Note: All videos created with the consent of the presenters. These are provided for personal research and study use only and may not be re-broadcast or copied or transmitted for any other purpose without written permission of the presenter and the Director of IC@L.
Upcoming Seminars
Stay tuned for announcements!
Past Seminars
Machine Learning for Emotion Prediction, Ideology Detection and Polarization Analysis using COVID-19 Tweets
Speaker: Dr. Sanjay Madria
Institution: Missouri University of Science and Technology
Date: August 18th, 2023
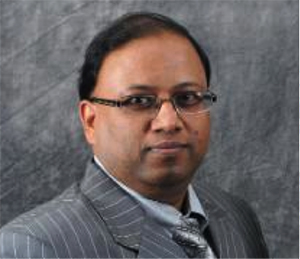
Abstract
The adversarial impact of the Covid-19 pandemic has created a health crisis globally all over the world. This unprecedented crisis forced people to lockdown and changed almost every aspect of the regular activities of the people. Thus, the pandemic is also impacting everyone physically, mentally, and economically, and it, therefore, is paramount to analyze and understand emotional responses during the crisis affecting mental health. Negative emotional responses at fine-grained labels like anger and fear during the crisis might also lead to irreversible socio-economic damages.
On the Relationship between Information Processing and Fitness in Biology
Speaker: Dr. Peter J. Thomas
Institution: Case Western Reserve University
Date: March 23rd, 2023
Abstract
Information transmission and storage have gained traction as unifying concepts to characterize biological systems and their chances of survival and evolution at multiple scales. Despite the potential for an information-based mathematical framework to offer new insights into life processes and ways to interact with and control them, the main legacy is that of Shannon’s, where a purely syntactic characterization of information scores systems on the basis of their maximum information efficiency. The latter metrics seem not entirely suitable for biological systems, where transmission and storage of different pieces of information (carrying different semantics) can result in different chances of survival. Based on an abstract mathematical model able to capture the parameters and behaviours of a population of single-celled organisms whose survival is correlated to information retrieval from the environment, our recent paper explores the aforementioned disconnect between classical information theory and biology. In this paper, we present a model, specified as a computational state machine, which is then utilized in a simulation framework constructed specifically to reveal the emergence of “subjective information”, i.e., the trade-off between a living system’s capability to maximize the acquisition of information from the environment, and the maximization of its growth and survival over time. Simulations clearly show that a strategy that maximizes information efficiency results in a lower growth rate with respect to the strategy that gains less information but contains a higher meaning for survival.
How to Haptic: Supporting Design of Haptic Interactions
Speaker: Dr. Karon MacLean
Institution: University of British Columbia
Date: March 7th, 2019
Abstract
Today’s advances in tactile sensing and wearable, IOT and context-aware computing are spurring new ideas about how to configure touch-centered interactions in terms of roles and utility, which in turn expose new technical and social design questions. But while haptic actuation, sensing and control are improving, incorporating them into a real-world design process is extremely difficult and poses a major obstacle to adoption into everyday technology.
In this talk I’ll focus on how my group has approached research into viable roles and design languages for physical communication by digging into a few divergent examples. Recently, these include:
- Affective physical human robot interaction: e.g. raising an interactive agent’s physically expressed ‘emotional intelligence’ by exploiting low-cost, stretchy touch sensing and dynamic pattern recognition, and easily-evolved and programmed mechanical platforms, where motion input and evaluation of its expressivity are tricky;
- Case study observations of novice and expert haptic designers (e.g., while building a haptic learning environment) to establish their challenges and needs
The broader question is how to support design. I’ll describe how we’ve built what we’ve learned about users’ cognitive and expressive frameworks from this and other research into a multitude of guidelines, tools and DIY systems, all available online, and our plans to push this into a broader openhaptics effort.
Capsule Networks
Speaker: Dr. Geoffrey Hinton
Institution: University of Toronto
Date: February 26, 2019
Abstract
I will describe a new type of neural net that uses small groups of neurons called capsules. A capsule outputs the probability that an instance of the kind of entity it detects is currently present but it also outputs a pose vector that represents attributes of the instantiated entity such as its precise position, orientation, scale, colour, deformation, motion etc. A capsule receives input vectors from lower-level capsules, transforms them with weight matrices, and looks for sharp agreement among a subset of the transformed vectors. It then produces an output vector that is the average of the transformed vectors that agree and an output probability that is determined by the sharpness of the agreement. This “Hough” non-linearity is very different from the ones standardly used in artificial neural nets and it has a very attractive property called “coincidence filtering”. For example, a capsule can recognize a familiar shape by detecting agreement between the predictions that different parts of the shape make for the generalized pose of the whole shape and it can completely ignore irrelevant predictions. This way of recognizing shapes generalizes naturally across changes in viewpoint because all the poses change in the same way so the coincidence remains. The output of a shape-detecting capsule is initially shared among many higher-level capsules to detect larger, more complex shapes. Higher-level capsules that converge on a tight cluster then demand a larger share of the output from the lower-level capsules that contribute to the cluster and smaller share from the lower level capsules that produce outliers. I shall argue that this “routing-by-agreement” is a much better way of routing each part of the visual input to those high-level neurons that know how to deal with it than “max-pooling”, which is the primitive routing mechanism currently used in convolutional neural nets. Stochastic gradient descent in a deep hierarchy of convolutional capsules that use adaptive routing should allow us to parse images using deep parts-based models that do not require hand-engineering.
Free software – your freedom in computing
Speaker: Richard Stallman
Institution: Free Software Foundation
Date: February 7, 2019
Abstract
Richard Stallman will speak about the goals and philosophy of the Free Software Movement, and the status and history of the GNU operating system, which in combination with the kernel Linux is now used by tens of millions of users world-wide.
Dimitris Metaxas – “AI and Model-based Methods for Robust and Scalable Biomedical Applications”
Speaker: Dimitris Metaxas
Institution: Rutgers University
Date: December 13, 2018
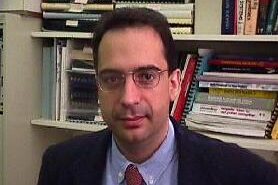
Centre for Innovation in Computing at Lassonde (IC@L) is a research unit focusing on the science of computing and its realizations to enable novel solutions and technologies. The centre hosts an annual Distinguished Speakers Series that invites notable computational scientists from academia and industry. A focus session is held in addition to the Speaker’s lecture. EECS grads and PhD’s meet the invited Speaker in an informal setting to discuss and ask questions on industry related issues in computational science and get to know more about the Speaker’s area(s) of research.
Conversational Machine Learning
Speaker: Tom Mitchell
Institution: Carnegie Mellon University
Date: October 15, 2018
Safe Learning in Robotics
Speaker: Claire Tomlin
Institution: University of California at Berkeley
Date: May 4, 2018
Computational Sustainability and Resilience
Speaker: Alan Mackworth
Institution: University of British Columbia
Date: April 12, 2018
Learning from Sounds and Images
Speaker: Antonio Torralba
Institution: Massachusetts Institute of Technology
Date: February 27, 2018
The Continuing Quest for Secure and Usable Passwords Video
Speaker: Lorrie Faith Cranor
Institution: Carnegie Mellon University
Date: May 16, 2017
AI Foundations for Data-Aware Business Processes Video
Speaker: Giuseppe De Giacomo
Institution: Sapienza Universita’ di Roma
Date: April 11, 2017
Demetri Terzopoulos – “The Computer Simulation of People: From Biomechanics to Intelligence”
Speaker: Demetri Terzopoulos
Institution: University of California
Date: January 10, 2017
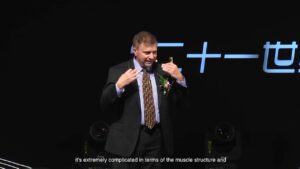
Model Based Vision for Robot Applications
Speaker: Henrik I Christensen
Institution: Georgia Institute of Technology
Date: April 8, 2016
Simulating a Functional Populace: From Steps to Stories
Speaker: Norman Badler
Institution: University of Pennsylvania
Date: March 18, 2016
Mining Large Graphs: Patterns, Anomalies, and Fraud Detection
Speaker: Christos Faloutsos
Institution: Carnegie Mellon University
Date: February 5, 2016
Realizing the Vision of Symbiotic Cognitive Computing Video
Speaker: Jeff Kephart
Institution: IBM Thomas J. Watson Research Center
Date: January 22, 2016
Has object vision been solved?
Speaker: Simon Thorpe
Institution: Université de Toulouse
Date: November 11, 2015