Lassonde grad student using AI to predict flooding in major urban centres
Tags:
Usman Khan, Assistant Professor in Civil Engineering, and Everett Snieder, a PhD candidate in Professor Khan’s group, have been working on machine learning techniques to predict when floods may occur. Predicting when floods may occur is one of the best methods to mitigate flood-related damage and costs.
One of the costliest natural disasters in Canadian history occurred in 2013 when Southern Alberta was flooded. Due to heavy rainfall, the Bow, Elbow and Highwood Rivers quickly overflowed, resulting in the displacement of hundreds of thousands of Canadians, several lives lost and billions of dollars in damages. The stress placed on the cities was enormous and Albertans are left dealing with the aftermath of the event to this day.
In 2013, Toronto was also faced with a significant flooding event when, in early July, significant rainfall overflowed waterways, backed up drains and flooded the Don River. Flooding causes immense damage to cities, businesses and homes which is why there is an increased focus and investment in improving flood mapping and flood forecasting.
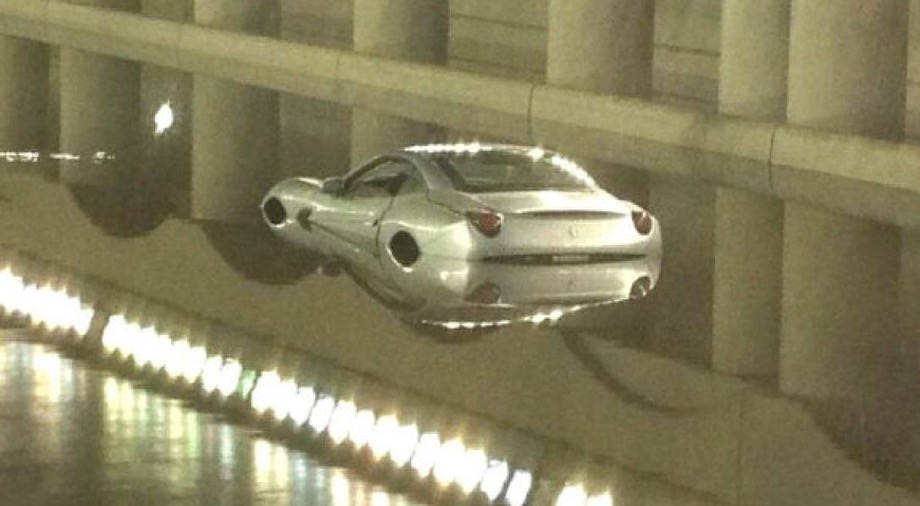
PhD candidate Everett Snieder, has been working on flood prediction for several years. As a PhD student in Professor Khan’s group, he was the project leader on a published 2019 study that developed models using artificial neural networks (ANN), a type of artificial intelligence, to predict when flooding would occur. This study focused on the Bow River in Alberta and the Don River in Ontario – two of the rivers which overflowed and caused damage in 2013. After completing this paper, Snieder identified that in some cases, the ANNs were unreliable in some of their predictions. Floods are a challenge for models, as they’re extremely rare, and predictive mechanisms tend to rely on the persistence of typical conditions. However, flood prediction is the rare case where anomalies are the focus.
“In general, models are trained to ignore outliers. In the case of flood forecasting, only about 1 observation in 1,000 is an actual flood. Therefore, we need to make sure that model training algorithms don’t ignore these points; they’re the ones we’re most interested in,” commented Snieder.
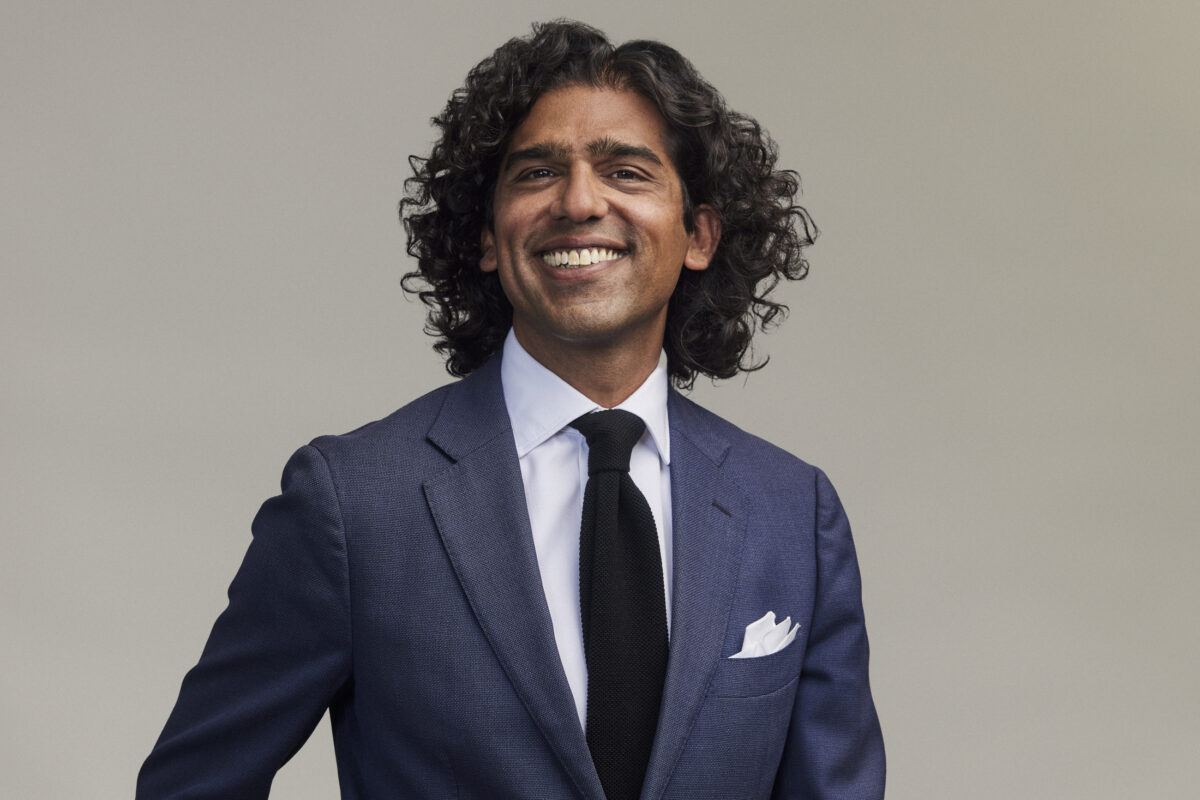
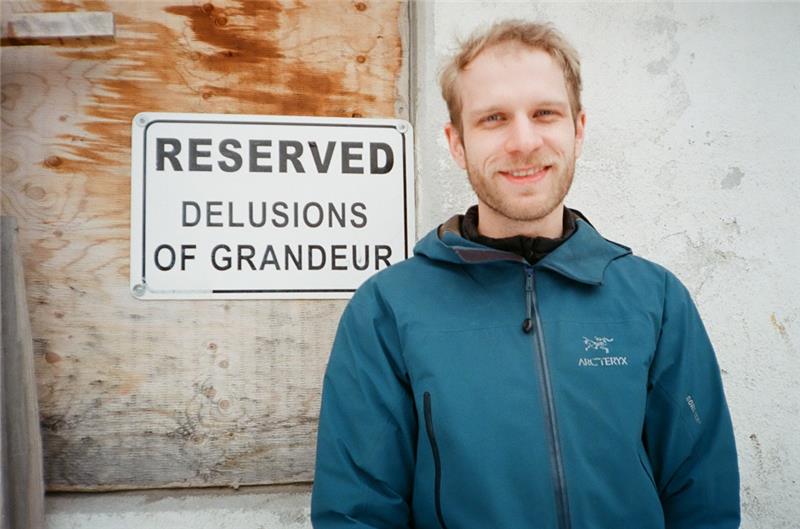
To counteract this effect, Snieder proposed and evaluated the use of various resampling and machine learning algorithms to boost the performance of ANN models on these infrequent flood flows. He found that modelling techniques that emphasized the flood data points improved the predictive capacity of ANN models. This work was recently published in Hydrology and Earth Systems Sciences (HESS), titled “Resampling and ensemble techniques for improving ANN-based high flow forecast accuracy”.
Developing these modelling techniques was not always an easy task for the Civil Engineering grad student. Snieder completed his undergraduate degree in water resources engineering and did not have a strong background in computer science or mathematics prior to joining Professor Khan’s group in 2017 as a master’s student.
“I ended up spending the majority of my masters just learning the code and material necessary to set up these machine learning models,” said Snieder. “I knew I wanted to come back to do my PhD. It was unfinished business. Additionally, Usman and I have a great relationship, he lets me pursue topics and research questions that interest me.”
Professor Khan decided to create a graduate-level reading course to help facilitate Snieder’s ascension to ANN and flood forecasting expert. This gave Snieder the opportunity to pursue a deep dive into various artificial intelligence algorithms and methods that could be utilized in his work. Snieder worked together with Karen Abogadil, an undergraduate research student supported by the Lassonde Undergraduate Research Assistant (LURA) program, who had begun working in Professor Khan’s group and expressed interest in AI-based applications.
Many AI-based techniques are popular in computer science research with limited applications in hydrology and Abogadil was instrumental in finding techniques that could be applied to the flood prediction models. Abogadil will be returning to Professor Khan’s group as a master’s student in Fall 2021.
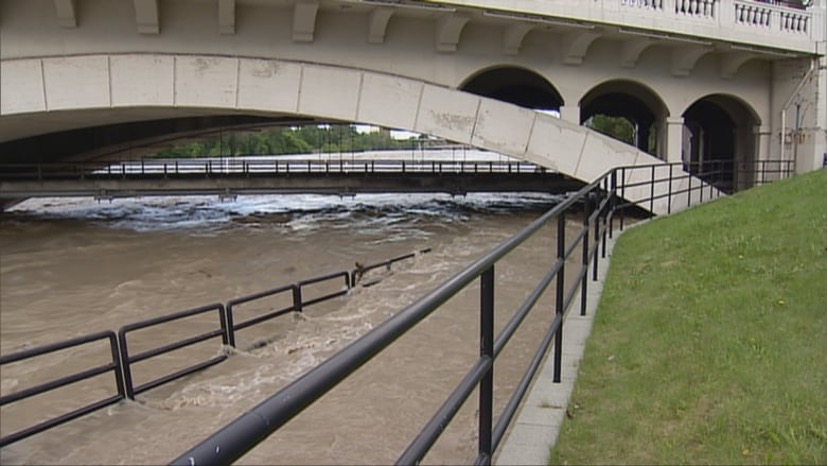
“There are numerous models that attempt to replicate the natural water cycle of evaporation, infiltration and precipitation but they all have their own complexity and issues with uncertainty,” commented Professor Khan. “This AI-based approach bypasses all these issues by just using raw rainfall data.”
Professor Khan’s team has demonstrated that AI applications for flood risk need to be added to the toolbox of methods used for flood forecasting, but their work is not done. They want to make the models even more robust. The team will be extending their work to include different classifications of algorithms along with modelling different rivers beyond the Bow and Don Rivers.
This story also appeared in YFile.