Lassonde prof. Khan presents new automated machine-learning approach for flood prediction
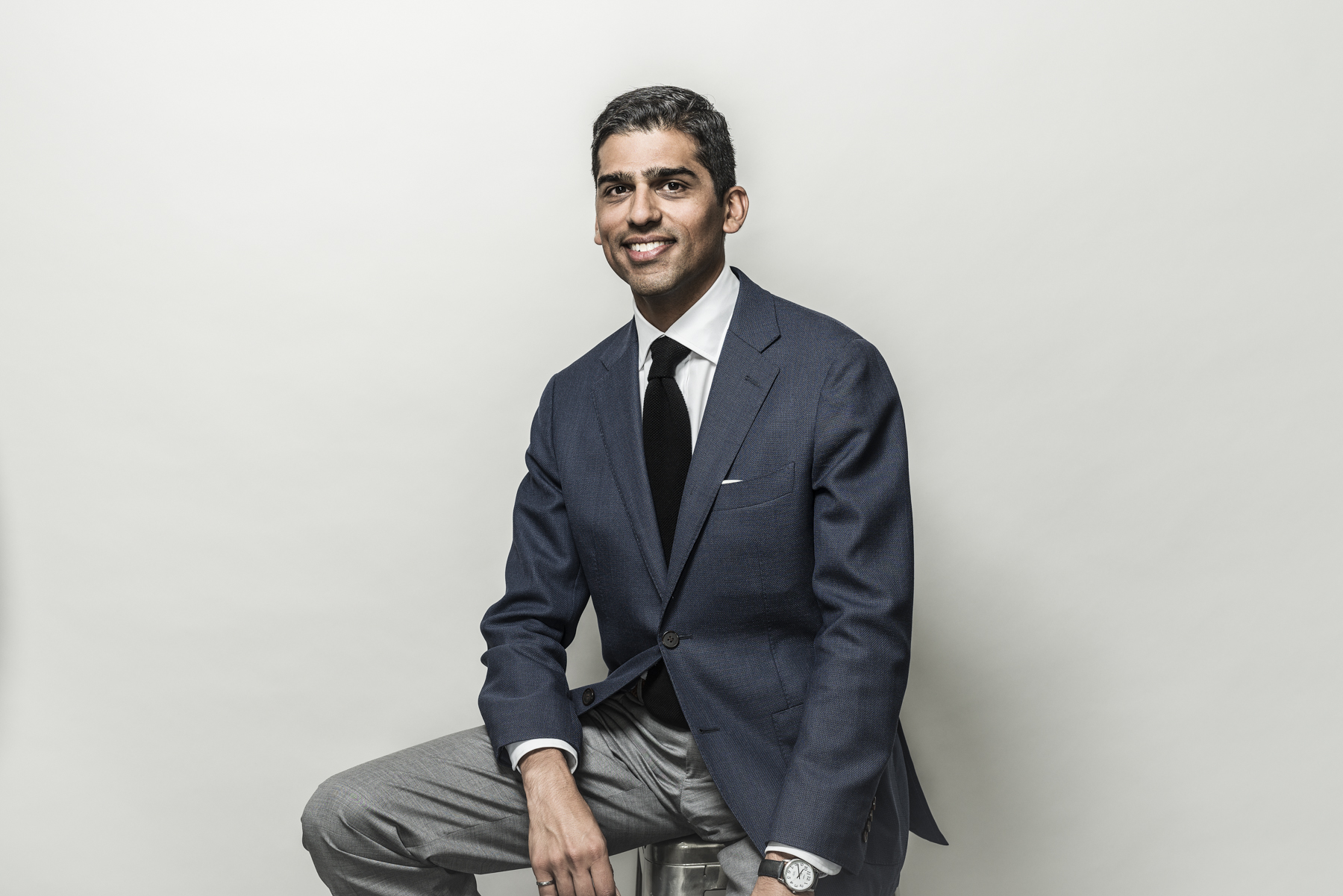
Lassonde Civil Engineering Professor Usman Khan’s paper, River flood prediction using fuzzy neural networks: an investigation on automated network architecture, has been published in Water Science & Technology.
The paper presents a new automated machine-learning approach for flood prediction in cities. The paper primarily covers the value of flood prediction and its importance for better flood management.
Kahn finds in his paper that machine learning methods show lots of promise in predicting floods accurately and promptly.
What makes this approach useful?
What is useful about this approach (using this measure of strength to select inputs) is that we can measure the power of the data on an annual (or seasonal) basis. The process can identify how the impact or relevance of each input changes with time, which can help us improve our understanding of the system. The dynamic nature of the input strengths can also capture changes in the system. For example, increasing urbanization might lead to more severe floods – this type of change might be measurable while looking at the input strengths. – Khan In general, what makes this process so attractive is its diverse application to any machine learning method, that is not specific to flood management. Most data-driven models have considerable uncertainty due to uninstructed input use. Suitably, this approach offers better processes for identifying such parameters.
Ultimately, Khan foresees his research model to be utilized by agencies like the Toronto & Region Conservation Authority, or other conservation authorities and municipal governments. The same approach can also be used by hydropower generation firms to improve their reservoir management systems.