Lassonde Professor Matthew Perras receives award from Government of Canada’s New Frontiers in Research Fund
Tags:
This article includes excerpts from an article originally published in YFile.
Matthew Perras, Assistant Professor in the Department of Civil Engineering, is one of four York University researchers that have received research awards from the Government of Canada’s New Frontiers in Research Fund (NFRF)-Exploration stream.
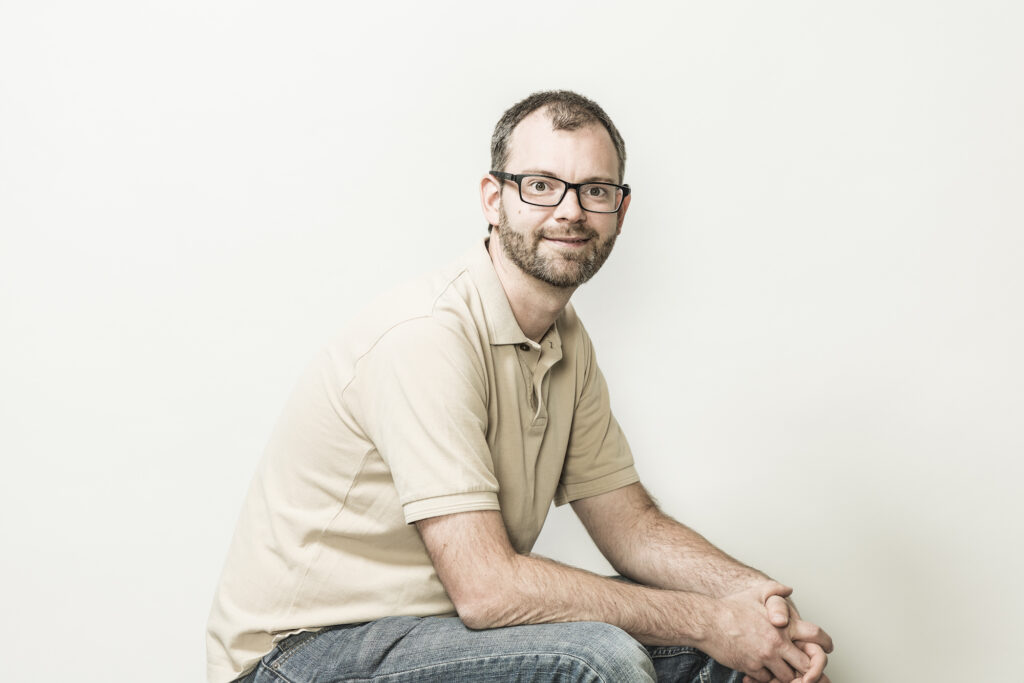
Dr. Matthew A. Perras, assistant professor, Department of Civil Engineering at the Lassonde School of Engineering is the principal investigator on the project “Using machine learning to understand ancient climatic influences on the stability of cliffs and tombs in the Theban Necropolis of Egypt.” Working with his colleague Dr. Usman T. Khan (Co-PI), also an assistant professor in the Department of Civil Engineering at Lassonde, and international partners Prof. Susanne Bickel (Co-applicant) from the University of Basel, Switzerland, Dr. Martin Ziegler (Collaborator) from ETH Zurich, Switzerland and Prof. Hesham Ahmed Hussin Ismaiel (Collaborator) from South Valley University, Egypt, the research focuses on the Theban Necropolis, a UNESCO World Heritage site comprised of tombs and temples near Luxor, Egypt. The project received $250,000 in funding.
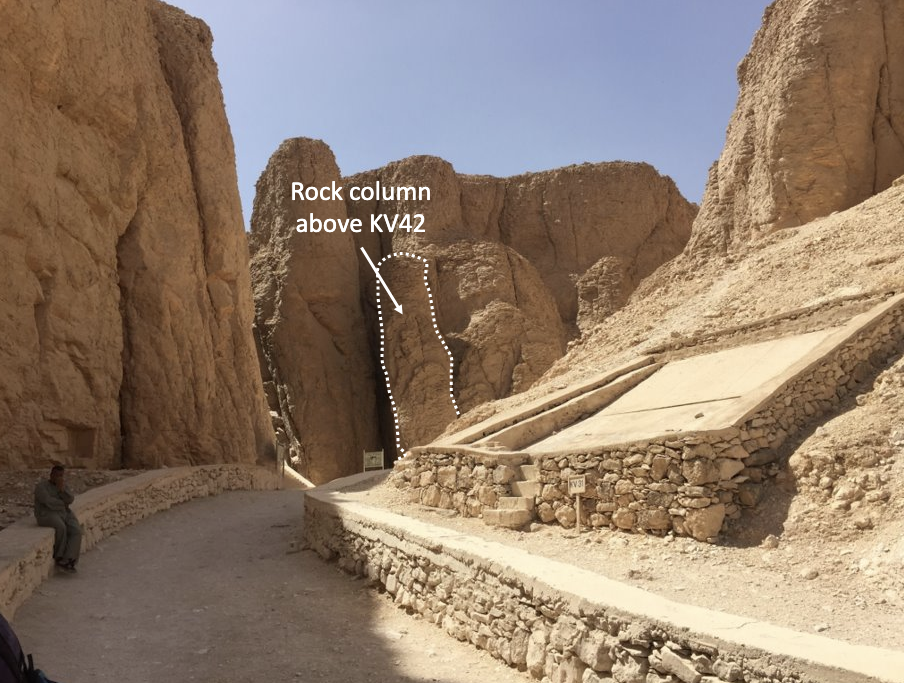
Most southern branch in the Valley of the Kings (KV). Several tombs of pharaohs from the New Kingdom (3500 – 3000 BP) were dug at the toe of tall (up to 30 m) and steep cliffs. Nowadays, the site is visited by tourists and archeologists. In the picture, the tomb KV42 is located at the back. Above its entrance, a 20 m tall rock column hangs onto a taller back cliff. The column is attached to the cliff by a 2 m tall rock bridge at its base.
The tombs in the Theban Necropolis are often shallow excavations with entrances at the base of cliffs and our archaeological team members have existing projects in the Necropolis that can be further investigated to understand their past stability, including Sheikh ‘Abd el-Qurna and the Kings Valley. The tombs hold evidence of rock mass collapses during construction through to recent deterioration leading to potential instabilities. Climatic variations are known to cause the rock to deteriorate, however, there is debate about the exact influence on crack growth rates. We lack detailed observations and experiments on long-term crack growth in rock since such experiments span many months or even years. In addition, current numerical tools are not capable of capturing the influences of climate change on crack growth. This leads to challenges in determining when instabilities will develop and to problems designing preservation strategies.
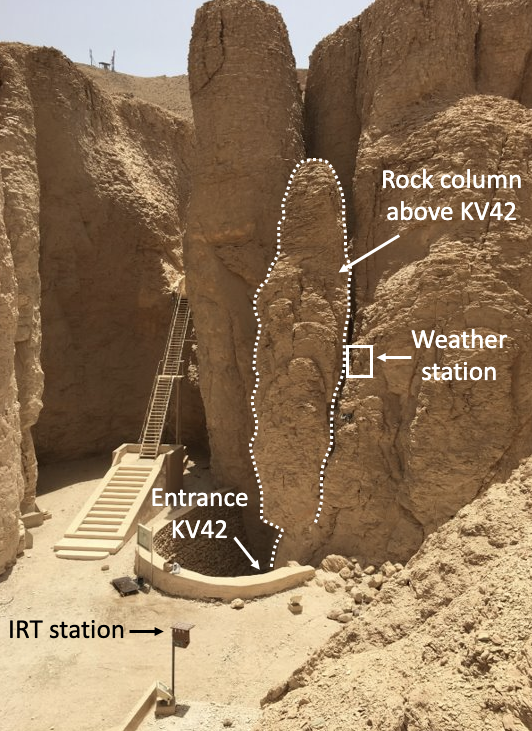
The rock column above KV42. The site has been instrumented to monitor the displacement from the back cliff, and the rock mass thermal response. The monitoring system consists of a weather station to measure climatic conditions (e.g. air temperature, solar radiation, wind speed, wind direction, relative humidity), which is also connected to a crack meter. In addition, an infrared thermographic (IRT) station captures thermal images to track the thermal response of shallow layers of the rock mass. The system has been recording data from April 2018 to date.
To address these challenges, Perras and the research team propose to utilize machine learning (ML) to analyze existing climate data and crack growth indicators to predict instability. An ML algorithm will be trained on current measurements (weather sensors & crack displacement sensors), then on historical climate data and photographs of crack growth. Ancient climate records and models (Nile sedimentation, tomb flooding & collapses) will be explored and could be used to back analyze the influence on crack growth on time scales yet to be studied.
With the expertise of geotechnical engineering, geology, archaeology, data and climate science, the researchers will seek to understand the prevailing conditions that led to the current state of stability and develop guidelines for preserving the stability into the future. The novelty of this research is in the combination of machine learning with archaeology and geological engineering.
Machine learning in both fields is in its infancy, however, such techniques allow for nuanced behaviours to be extracted from large and complex data sets as in this project. Understanding the current measurements, past influences – both historical and even ancient – and applying them to predict future instabilities will help to identify key areas for protection and aid in preserving this UNESCO World Heritage site for generations to come.