Machine learning makes waves: Novel model helps advance flood risk management research
Tags:
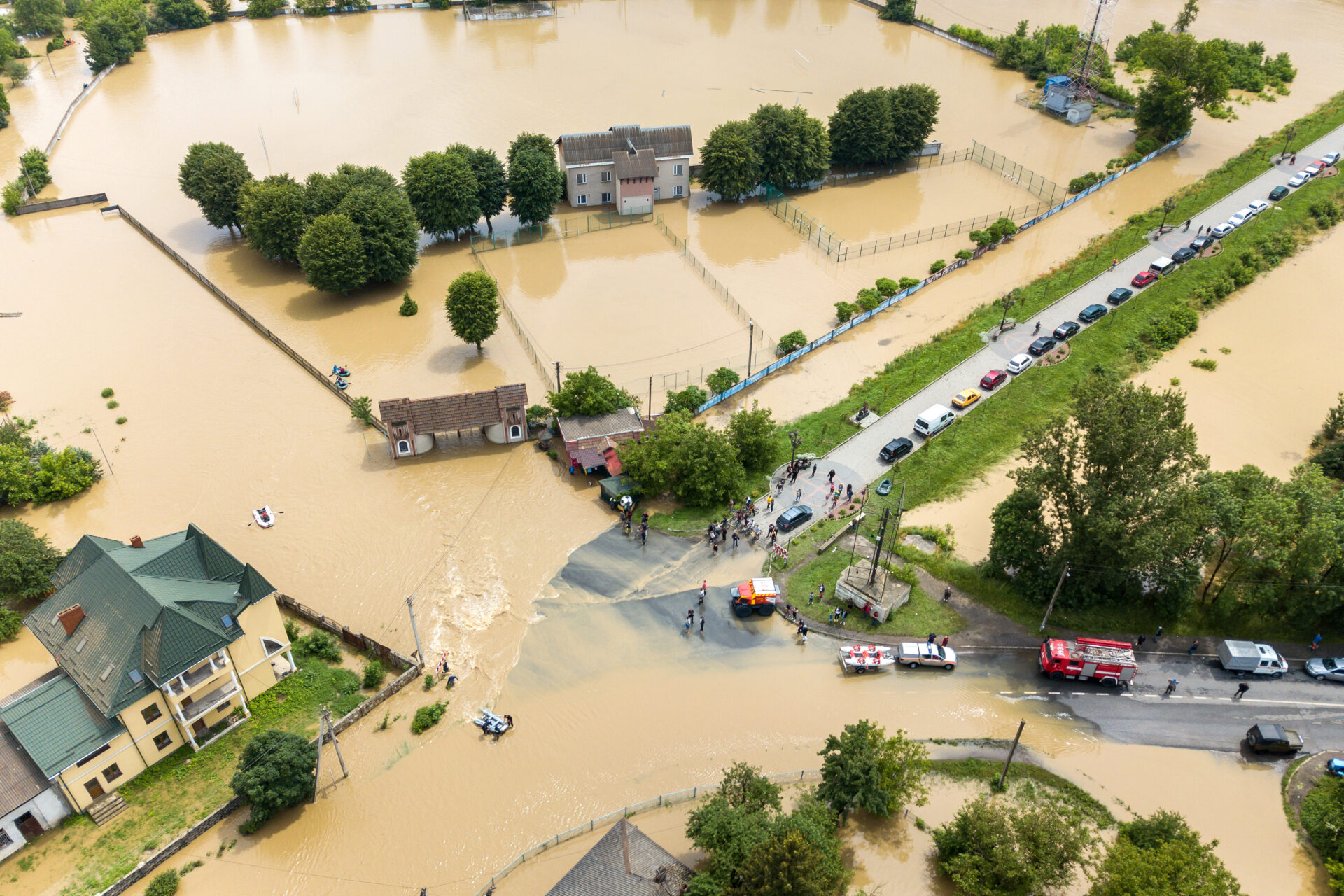
In a recently published paper, Rahma Khalid, PhD candidate in the Civil Engineering department at York University’s Lassonde School of Engineering and her supervisor Usman Khan, associate professor, proposed a promising new model for flood susceptibility mapping (FSM) which incorporates machine learning (ML) methods.
Flood susceptibility mapping is a valuable technique used to identify areas that are vulnerable to flooding and inform risk mitigation and protection strategies. Unfortunately, conventional FSM methods rely on time-consuming physical and mathematical models that are also limited in their ability to predict flood risk across large regions.
“We have seen that physical and mathematical models can be very inconvenient for flood susceptibility mapping, especially when it comes to analyzing large areas,” says Khalid. “From a research perspective, we know that using machine learning can improve the speed and efficiency of different processes. This is why we proposed a flood susceptibility mapping model that is leveraged by machine learning for more accurate, rapid and reliable results.”
Putting their idea to the test, Khalid and Professor Khan utilized an ML model to map out different regions in Southern Ontario and determine their flood susceptibility. The model performance was also compared against conventional physical and mathematical models, as well as various emerging ML methods.
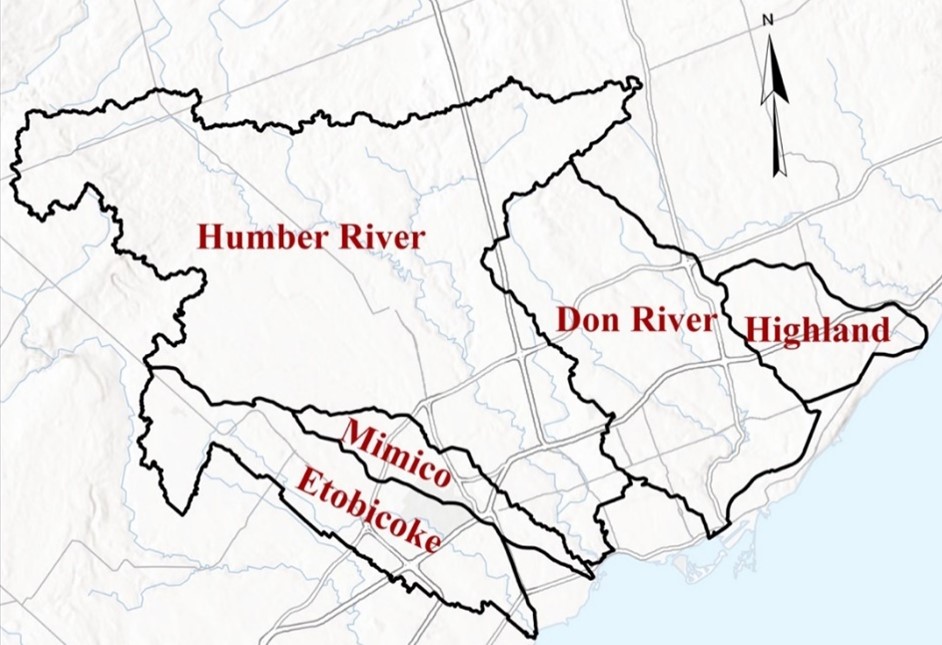
“When it comes to flood susceptibility mapping in real-world scenarios, machine learning models have not really been used,” says Khalid. “Industry members are also hesitant to apply these models because there is very little information about their accuracy and reliability.”
The proposed model utilizes an Artificial Neural Network – Synthetic Minority Oversampling Technique (ANN-SMOTE) ensemble, which exhibits well-rounded characteristics and addresses limitations of other FSM models. Like a student preparing for an exam, the ANN-SMOTE ensemble was trained with previously-gathered data from different regions across Southern Ontario, allowing the model to interpret, identify and predict areas that are at risk of flooding.
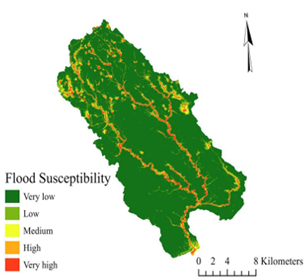
After training and testing, the ANN-SMOTE ensemble proved to be a superior method for flood susceptibility mapping, outperforming other models while demonstrating novel capabilities that can help advance the future of flood risk management.
“Our model demonstrated a novel ability to accurately predict flood susceptibility, even across areas that we did not provide training data for,” says Khalid. “Knowing this, we can work towards training our model to understand more about different regions and further improve its ability to predict flood susceptibility in larger areas.”
Currently, Khalid and Professor Khan are working on enhancing the performance of the ANN-SMOTE ensemble. They are particularly focused on improving data resolution and exploring the possibility of supplementing their model with additional ML methods.