All Aboard! Next Stop, the Autonomous Train
Tags:
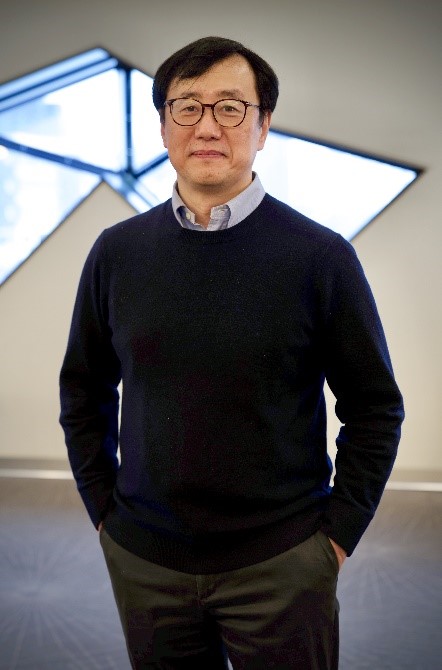
When you think of autonomous vehicles, what usually comes to mind are self-driving cars (such as those made by Tesla), and the significant research efforts being undertaken to ensure this technology is road-ready. However, there is another form of transportation where autonomous technology adoption not only poses its own set of domain challenges, but more importantly, has the potential to significantly improve a nation’s ability to transport both passengers and freight. This of course, is rail transport, and through his research at the Lassonde School of Engineering at York University, Professor Gunho Sohn is working towards ushering in the new era of the autonomous train.
With funding from the Ontario Vehicle Innovation Network (OVIN), Sohn’s team collaborated with Thales Canada and Lumibird Canada to create and test a system to enable autonomous rail operations. His group’s expertise in computer vision and machine learning was combined with Lumibird’s LiDAR technology to create an object classification, detection and tracking system that was integrated into Thales’ controls module and tested in real-world conditions.
While driverless transit systems already transport millions of riders worldwide each day with the utmost safety, reliability and performance, these systems are only automated, not autonomous. That means, they only repeat set movements within controlled environments under centralized control.
“Driverless automated trains rely on heavy deployment of infrastructure such as wireless communication systems to position trains and control navigation,” says Sohn. “However, infrastructure may malfunction, position is lost, and the entire train stops until a human operator intervenes.” The crucial difference is that an automated trains lack the capability to dynamically sense its local surroundings and make intelligent decisions from this information on its own. This sensing system is precisely what Dr. Sohn and his team of researchers have been working on over the last 30 months.
Their first challenge was to collect and generate the millions of data points needed to represent static objects such as buildings, and moving objects such as cars, humans or animals that may come onto the tracks. A combination of cameras and LiDAR were incorporated into the sensor architecture. One camera with a short focal length and wide angle of view detects objects close to the train; another camera with a longer focal length detects objects at a distance; and finally LiDAR, a laser-based perception sensor, handles the scenarios where cameras may perform sub-optimally including during night time and under adverse weather conditions. “All of these components augment each other, what one misses, the other detects,” emphasizes Sohn. “Therefore, a large part of this work was studying the interplay between the different systems”.
How does computer vision detect and classify surrounding buildings or people jumping onto rails, or distracted cyclists or drivers crossing the tracks? “Exactly the same way human vision works in deriving information,” explains Sohn. “For example by colour, shape, contrast, saliency and other visual cues, and by context such as the spatial relationship between objects.”
Another important aspect of the work was to detect and model the train tracks using camera and LiDAR in various illumination conditions. To know if anything is impinging on it, you fundamentally need to know where the track is at all times. Furthermore, autonomous trains would need to anticipate upcoming railroad switches to adjust speed and obtain authorization to safely proceed.
With the sensor architecture and perception module developed, a 16 km-long track at York Durham Heritage Rail (YDHR) was used to validate their system under a variety of typical and adverse scenarios. The trials were a success and the project just concluded in December 2021.
While certainly challenging, this work has also been quite rewarding for the Lassonde team. “This has been one of the best projects in my academic life,” enthused Sohn. “The field of robotics and AI is very resource-intensive, and developing an end-to-end prototype presented a rare training opportunity for students.” During the project, Thales provided internships to three doctoral students, and at its conclusion, two postdoctoral fellows were hired by Thales’ Autonomous Group.
Even with patents and publications from this project pending, Sohn and his team are already looking ahead to the next phase. They are continuing the work with Thales focusing on autonomous safety, securing funding from MITACS and ScaleAI, bringing onboard fellow Lassonde Professor Sunil Bisnath to integrate GPS, and exploring specific applications such as freight transport with several Toronto-based AI companies.
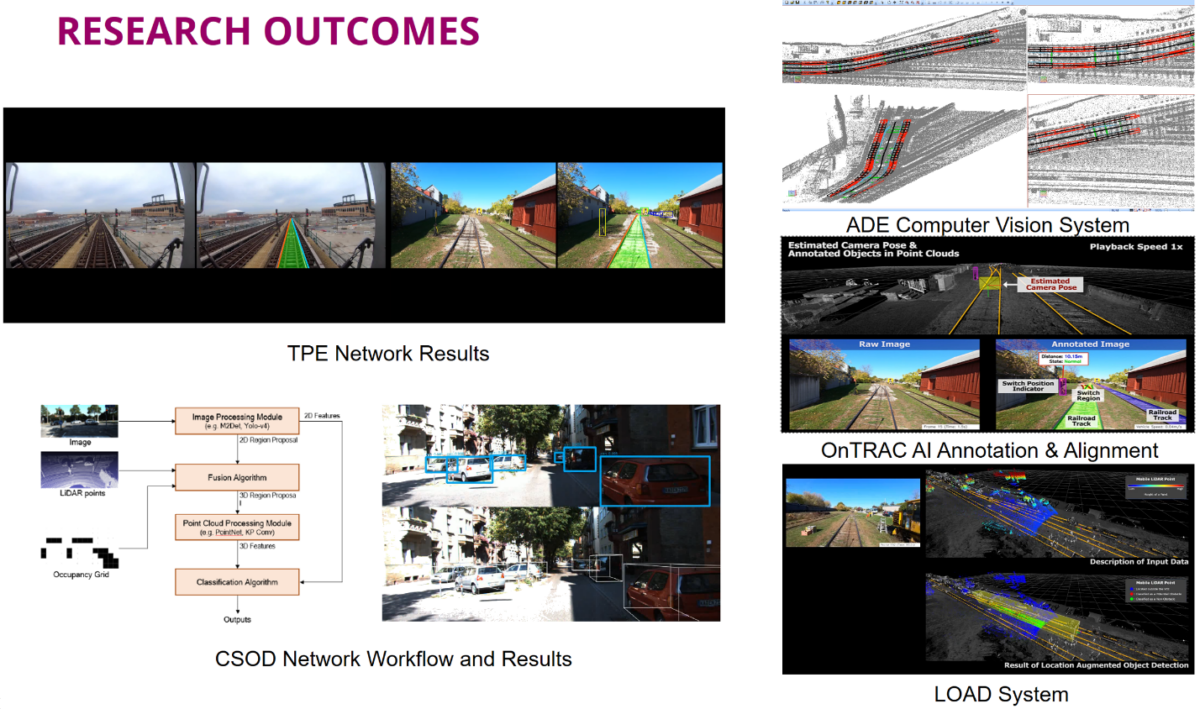
This work is part of a multi-faceted research program that reflects sustainable and autonomous transportation as a growing strength at the university. Under Sohn’s leadership, a cluster of researchers in disciplines ranging from Civil Engineering to Electrical Engineering and Computer Science, are tackling future transportation challenges using AI approaches, positioning the Lassonde School of Engineering and York University as the next hub for innovation in this field.