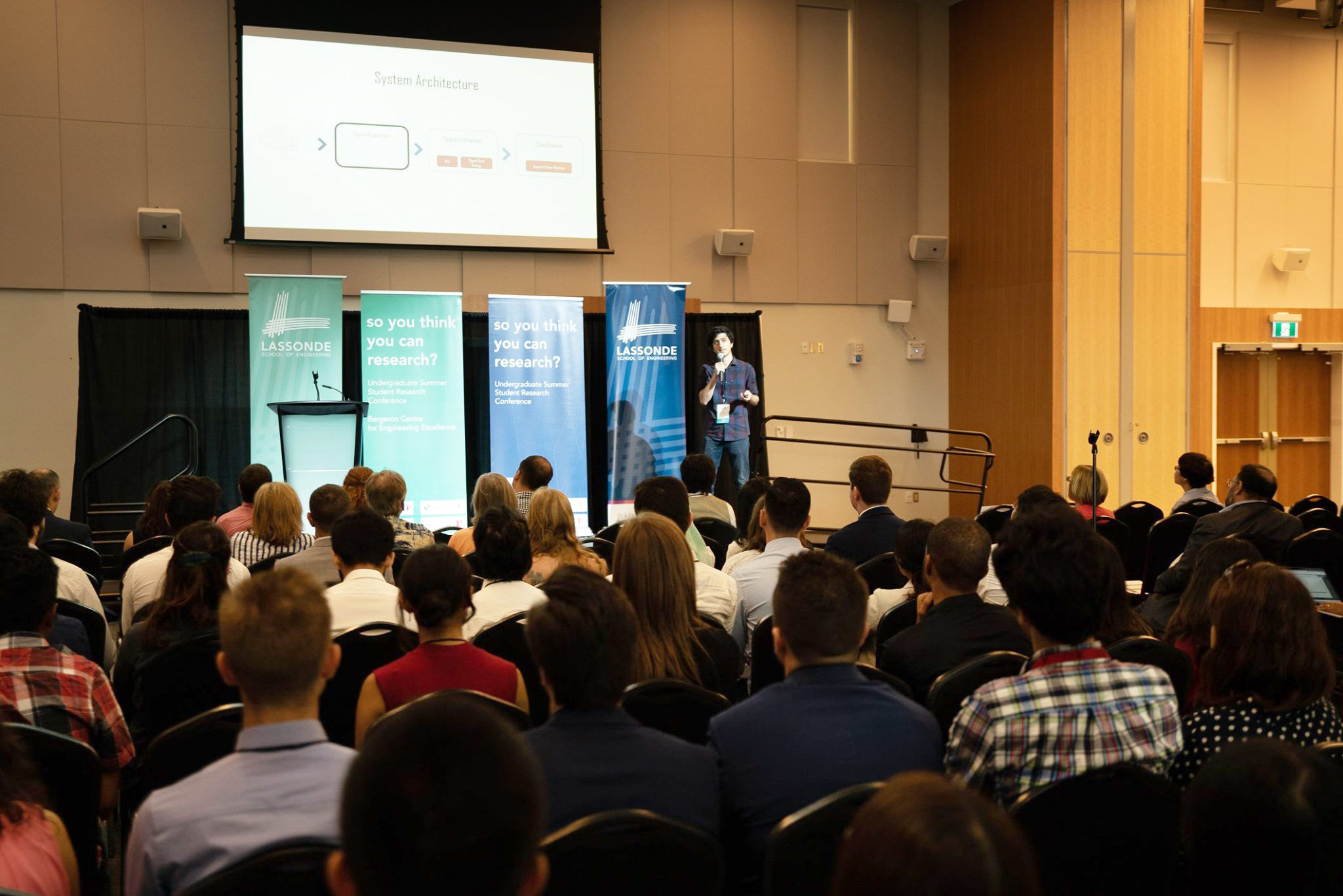
Lassonde Undergraduate Summer Research Conference 2024 Researchers
Get to know your researchers!
Project Presentations
This year’s research conference includes project presentations of undergraduate students and their research experiences. Read firsthand as these students share their experiences and discuss the various research opportunities available to undergraduates. Explore a variety of topics and subject matter at this year’s conference. Learn about recent discoveries, cutting-edge research, and emerging technologies in fields such as finding bugs in Java code, removing Fluoxetine from wastewaters, Metaverse-enhanced pedestrian safety, microplastics detection in wastewaters and sludges, reinforcement learning algorithms, and many more.
Researchers
-
Civil Engineering
Jason Wade
Program: Mary & Hubert Lynch Undergraduate Research Internship
Supervisor: Magdalena Krol and Usman Khan
Abstract Title: Exploring the Efficacy of Hydrological Performance of Green Roofs
Abstract Authors: Jason Wade, Sebastian Ceron, Karen Dunbar, Magdalena Krol, Usman Khan
Abstract: Green roofs are a building technology that are commonly installed in urban areas to retain and detain rainwater. This study aims to directly compare the hydrological performance of a green roof module with a conventional roof module located at York University in Toronto, Ontario. The green roof design will use commercially available modular units installed on roofing material, while the conventional roof design will be a commercial flat roof. The two different designs will be instrumented to measure surface runoff, and in the case of the green roof design, drainage, storage, and evapotranspiration. A nearby weather station will collect relevant meteorological data. The collected data will be used to compare stormwater retention, detention, and peak runoff of the different designs. Preliminary results from our study will be discussed in the presentation where the retention and detention in the green roof design, as well as a reduction in peak runoff will be discussed for both roofs. This study is significant as it directly compares a green roof and conventional roof in the same location, clearly outlining benefits of using green roofs in urban settings.Mustafa Abdulkadhim
Program: USRA
Supervisor: Satinder BrarShahrzad Rahmani
Program: LURA
Supervisor: Satinder Brar
Abstract Title: Biofilm Formation on Microplastics in Wastewater: Factors and Implications
Abstract Authors: Shahrzad Rahmani, Mustafa Abdulkadhim, Gaurav Bhardwaj, Satinder Kaur Brar
Abstract: Microplastics (MPs) pose a complex challenge in contemporary wastewater treatment plants (WWTPs), particularly in biological treatment systems. MPs present a unique interface for biofilm formation. This biofilm formation potentially alters MPs’ properties, impacting their transport and fate in WWTPs. This study aims to explore MP properties as an influencing factor for biofilm formation and evaluate the subsequent impact on treatment performance. Methodologically, the study employs spiking five different MPs; Polyethylene (PE), polypropylene (PP), Polyethylene Terephthalate (PET), and Polylactic Acid (PLA) in mixed liquor, enriched with synthetic wastewater, for 4 weeks at 25°C, 150 rpm. The biofilm biomass was quantified using crystal violet (CV) assay and Scanning electron microscopy (SEM) to explore the biofilm dynamics qualitatively. COD and Nitrogen removal were assessed for treatment efficiency while EPS was quantified to assess the effect of microplastics (MPs) on microbial communities. The preliminary results indicate that PLA being a biodegradable polymer supports higher biofilm growth as compared to others, negatively impacting the treatment efficiency initially, which recovered gradually, potentially linked to biofilm development. It was also found that the presence of MPs leads to increased EPS production in mixed liquor (ML). The study concludes that microplastics, particularly biodegradable PLA, significantly influence biofilm formation and EPS production, initially affecting treatment efficiency in WWTPs. These findings contribute to achieving SDG 6 (Clean Water and Sanitation) and SDG 14 (Life Below Water) by enhancing our understanding of MP impacts on wastewater treatment processes.Pouya Moradjounamin
Program: LURA
Supervisor: Shooka Karimpour
Profile: Pouya, a final-year Civil Engineering student is set to graduate next year. Currently serving as a Research Assistant at the Lassonde School of Engineering, he has chosen to participate in this research to gain practical experience and contribute to innovative engineering solutions. His passion for civil engineering drives his commitment to both studies and research endeavors.
Abstract Title: Use of Imaging in the Detection of Microfibers
Abstract Authors: Pouya Moradjounamin, Shooka Karimpour
Abstract: The detection and analysis of microfibers in various environments are critical for understanding their behavior and impact. This research leverages advanced imaging techniques, specifically Particle Image Velocimetry (PIV) and Particle Tracking Velocimetry (PTV), to study the dynamics of microfibers in diverse scenarios. By employing both PIV and PTV systems concurrently, we aim to obtain a comprehensive understanding of microfiber motion and distribution. In this study, we explore the interactions of microfibers under different flow conditions and environmental settings. The PIV system is utilized to capture high-resolution flow fields, allowing for the detailed observation of fluid dynamics and microfiber dispersion patterns. Concurrently, the PTV system tracks individual microfiber movements, providing insights into their trajectories and velocities. Our findings indicate significant variations in microfiber behavior based on flow characteristics and environmental parameters. The integration of PIV and PTV techniques has enabled us to correlate the fluid dynamics with microfiber trajectories, offering a holistic view of their behavior. This research contributes to the broader understanding of microfiber pollution and its implications, providing essential data for developing mitigation strategies. This research contributes to the broader understanding of microfiber pollution and its implications, providing essential data for developing mitigation strategies. By comprehensively analyzing the behavior of microfibers, we can inform better waste management practices and policies aimed at reducing microfiber contamination in aquatic environments. Moreover, the methodology developed in this study can be applied to other types of particulate pollutants, enhancing its relevance and applicability. Overall, the use of imaging technologies such as PIV and PTV in detecting and analyzing microfibers represents a significant advancement in environmental monitoring. The outcomes of this research will aid in formulating effective policies and practices to address microfiber pollution, aligning with the United Nations Sustainable Development Goals (UN SDG) of promoting clean water and sustainable industrial practices.
Rahil Aggarwal
Program: LURA
Supervisor: Satinder Kaur Brar
Profile: Rahil is a first-year engineering student who is pursuing civil engineering. He is enthusiastic and passionate about his work and enjoys being busy. He likes to help others and learn something new every day. He chose to participate in the research because he wanted to expand his knowledge in the field of environmental civil engineering and get hands-on experience since this is his first-time doing research.
Abstract Title: Robust Detection and Characterization of Microplastics in Wastewater
Abstract Authors: Rahil Aggarwal, Juviya Mathew, Satinder Kaur Brar
Abstract: Microplastics (MPs) (plastics < 5mm) are ubiquitously spread in water environment raising global concerns due to their low degradation rate and adverse effect on the nature. MPs enter wastewater treatment plants (WWTPs) via domestic and industrial wastewater (WW). To date, many studies have been conducted for MPs’ analysis in WW. However, all these lacked a standard protocol for laboratory scale detection and characterization of MPs in WW. Moreover, lack of unified data leads to difficulties while comparing and regulating standards to mitigate MPs’ population. This research addresses this gap by focusing on the primary and most crucial step in the MPs analysis i.e. pre-treating WW to remove suspended organic matter and extracting MPs for analysis. Using an innovative pretreatment method called “Ferro-sonication (Fe-UlS),” the suspended organic matter in WW, which can potentially interfere with MPs analysis, was efficiently removed at a lower temperature and lesser time. Samples from secondary treatment stage of WWTPs were used and solids analysis was performed with major focus on the total suspended solids (TSS). Polyethylene (PE) and Polypropylene (PP) MPs were then spiked in these samples and analyzed for physical, chemical, and thermal properties after Fe-UlS to study the effects of pretreatment on the inherent properties of these MPs. This was achieved using advanced analytical instruments such as stereomicroscope, Fourier Transform Infrared Spectroscopy (FTIR) and Differential Scanning Calorimetry (DSC). Fe-UlS reduced more than 85% of the TSS in secondary influent and effluent at 47°C in 30 minutes. PE and PP MPs were found to be stable, both physiochemically and thermally. The innovated pretreatment approach can be effectively used for both qualitative and quantitative laboratory scale MPs analysis in WW matrix. This helps in identifying the type, size, and shape of MPs and thereby becomes easier to track the origin of MPs into the WW matrix.
Samreen Sran
Program: USRA
Supervisor: Satinder Kaur Brar
Profile: Samreen is a biotechnology student in her final year and has a keen interest in bioremediation. When the opportunity arose, she eagerly applied to work in Dr. Brar’s Lab. This decision was driven by the lab’s focus on developing novel strategies to treat contaminants in water and wastewater, especially via bioremediation, an environmentally safe and sustainable solution. Additionally, she sought hands-on research experience and the chance to work in an inter-disciplinary lab environment to enhance her practical skills as well as knowledge in the field.
Abstract Title: Effective Removal of Fluoxetine Using Waste-Derived Biosorbent
Abstract Authors: Samreen Sran, Pratishtha Khurana, Satinder K. Brar
Abstract: Due to stressful lifestyles and modern medicine, there has been a steady but significant increase in the production and consumption of antidepressant drugs in the past few decades. Among several classes of drugs that are prescribed for the treatment of psychiatric and depressive disorders, selective serotonin reuptake inhibitors (SSRIs), particularly fluoxetine is one of the most prescribed drugs in North America. However, its consequent discharge into the aquatic environment both via human waste and disposal from hospitals and pharmaceutical industries has posed a major ecotoxicological concern owing to its potential to cause chronic toxicity and endocrine disruption in aquatic environment organisms. Since conventional wastewater treatment plants (WWTPs) are unable to efficiently remove fluoxetine, it is imperative to research sustainable and environment-friendly methods that can do so. This study focuses on the removal of fluoxetine via its adsorption on agri-food waste derived biochar. Adsorption is widely employed for the removal of many toxic pollutants from water as it is economical, sustainable and efficient. The biochar was produced from pyrolysis of olive stone, a by-product in the production of olive oil, followed by CO2 activation. The produced biochar was characterized using Fourier-transform infrared spectroscopy (FTIR) for surface functional groups, elemental analyser, and Brunauer – Emmett – Teller (BET) for surface area analysis. Different amounts of biochar were added to 50 mL solutions containing 50 mg/L fluoxetine (FLX) and removal was estimated by measuring concentration of fluoxetine over time. Removal efficiency greater than 80% was achieved within 2 hours with an adsorption capacity of > 100 mg/g. The findings suggest that adsorption onto biochar is an effective, low-cost approach for removing fluoxetine from water and highlight that valorization of waste into an adsorbent has an important application in environmental remediation.
Sebastian Ceron
Program: LURA
Supervisor: Magdalena Krol, Usman Khan
Profile: Sebastian is a 2nd year Civil Engineering student who chose to be a part of a research project because he wanted to explore his interests in research and see if he wanted to continue this in graduate studies. It is impactful as well, as Sebastian can increase his knowledge of new topics that will help him in his future. He sees research as being a skill development project, where he can improve his critical thinking, problem solving, and technical skills. Sebastian is excited to participate in the LURA program and make a contribution to the future of the research project.
Abstract Title: Comparing the Thermal Performance of Green and Conventional Roof Design
Abstract Authors: Sebastian Ceron, Jason Wade, Karen Dunbar, Magdalena Krol, Usman T. Khan
Abstract: In developing cities, urbanization continues to grow along with new buildings and paved spaces that contribute to the increase in heat due to the urban heat island effect (UHI). Green roofs (GR) are one solution to combat this issue. This study focuses on the thermal properties of a gr and conventional roof (CR) by developing and constructing two different types of roof modules at the york university campus in toronto, ontario. Since the climate zone is warm to hot summers and cold winters, this study focuses on a variety of weather patterns. Both modules were designed to have the same bottom layers, while the gr has the additional needed vegetation components. Fibre optic sensors were placed throughout layers to measure temperature differences. Sensible, latent, and ground heat flux were measured, which represent convection, evapotranspiration, and conduction respectively and inward and outward shortwave and longwave radiation were also considered. Additionally, an energy balance model was developed to consider the different heat fluxes in different areas of the roof. Preliminary results from our study will be discussed in the presentation examining if the gr reduced heat flux through the buildings, due to the additional insulation from substrate depth and vegetation and was able to provide a passive cooling effect due to evapotranspiration. This type of research is beneficial to understanding the thermal properties of implementing gr in urban cities and how different climate zones can affect the functioning of a GR.
Shannon Fernando
Program: USRA
Supervisor: Matthew Perras
Profile: Shannon is a graduating Atmospheric Science student interested in the
effects of climate change on Egyptian Sites. She hopes to return in Fall 2024 for her master's to continue her summer research. Shannon works part-time at The Weather Network and has a keen interest in both climate and weather, specifically in how they affect people.
Abstract Title: Compiling the Impact of Climate Variations in the Modern and Ancient Area of Luxor, Egypt
Abstract Authors: Shannon Fernando, Neil Tandon, Matthew Perras
Abstract: In recent years, the topic of climate change has garnered increasing attention as a rapidly advancing area of study, consistently demonstrating its significant impacts on various facets of both the environment and society. Variations in climate variables such as temperature, humidity and precipitation can lead to changes in ecosystems, weather patterns and even impact our built infrastructure. These variations can be studied uniquely in Luxor, Egypt, where climate change threatens the degradation of archaeological sites and ancient monuments. Understanding the complex relationships involved in climate change is essential in developing effective policies and strategies to mitigate the impending challenges. This research study investigates the climate trends from 1980 to 2023 to better understand the temporal variations in climate by utilizing hourly meteorological airport and modelled (meteoblue) data. Compared to previous research, this study offers a more localized and detailed understanding of the direct impact of climate change on Luxor. An algorithm was developed to organize the data, laying the groundwork to test the soundness of the data and the effectiveness of interpolating missing values. The analysis showed that Luxor is experiencing an increase in temperature but a decrease in relative humidity, in line with physical expectations. Here we show that the most significant average temperature increase was observed during august, with an increase of approximately 0.617°c per decade since 1980. Additionally, the most significant average relative humidity decrease was observed in November, with a decrease of approximately 1.334% per decade. These trends indicate that Luxor's already dry and hot climate is becoming both hotter and drier. These results reinforce the pressing need for mitigative and adaptive measures to protect Luxor's cultural heritage and infrastructure.
Yisroel Rosenberg
Program: LURA
Read More About the Conference
Supervisor: Magdalena Krol
Profile: Yisroel is currently in his 4th year of civil engineering. He is a motivated individual who is set on making impactful differences in our world. He chose to participate in research this summer in order to facilitate future environmental endeavors to make our world a better place for generations to come.
Abstract Title: Swelling Dynamics of Bentonite Under Repository Conditions
Abstract Authors: Yisroel Rosenberg, Aditi Chowdery, Farhana Chowdhury, Magdalena Krol
Abstract: Several nations, including Canada, Finland, Switzerland, and the United States, are exploring long-term strategies for storing used nuclear fuel. A prevalent approach involves the implementation of Deep Geological Repositories (DGRs), which are designed to be situated several hundred meters below the earth's surface. These DGRs will contain Used Fuel Canisters (UFCs) typically encased in multiple engineered barriers, each serving a specific purpose within the repository. In Canada, the Nuclear Waste Management Organization (NWMO) oversees the design and deployment of the DGR. The current NWMO design features a steel container coated with copper to act as a corrosion barrier, surrounded by highly compacted bentonite (HCB) and bentonite gapfill. Bentonite, a clay known to swell when in contact with water, is critical as it can impede the movement of corrosive agents toward the UFC, thus preventing canister corrosion. This research project aims to examine the swelling behavior of the bentonite gapfill under various repository conditions through laboratory experiments to assess the effectiveness of bentonite as a barrier against the migration of potentially corrosive substances. This will be achieved by analysing the gapfill material in powdered and granular forms within synthesized groundwater to determine the swelling potential of each form under DGR conditions. Groundwater can significantly affect the swell index of bentonite due to the presence of various dissolved ions. When bentonite comes into contact with groundwater, the ions can influence its swelling behavior by interacting with the clay particles. For instance, solutions with high levels of salinity can lead to a reduction in swelling capacity. This research will help provide more critical insights to ensure the long-term safety and integrity of Canada’s DGR.
-
Earth & Space Science and Engineering
Brock Koch Nichol
Program: Mary & Hubert Lynch Undergraduate Research Internship
Supervisor: John Moores
Profile: Brock is entering his 3rd year of Space Engineering. He is a curious learner and is passionate about technology, astronomy, and much more. Brock chose to participate in research to experience where the worlds of engineering and science merge together into innovation.
Abstract Title: Advancing Methane Spectroscopy Technology and Modeling Atmospheric Scattering with Lasers
Abstract Authors: Brock E. Koch Nichol, Kevin M. Axelrod, Alex C. Innanen, Charissa L. Campbell, John E. Moores
Abstract: Detecting methane on planetary bodies could be an indication that life may exist there. Methane has been observed on mars, however little is known about the origins and cycles of the gas in the martian atmosphere. Technology developed by abb inc. Known as integrated cavity-enhanced optical spectroscopy (icos) could be used to discover more about daily and seasonal variations in methane levels on mars, as well as measure the carbon 12/13 isotopic ratio to determine if the methane was produced from living organisms. Currently, the technology readiness level (trl) prevents the icos from being used on space missions. In part, the mars atmospheric gas evolution (mage) project aims to increase the trl by testing the icos in a series of laboratory experiments, a mars analog field site in the arctic, and a stratospheric balloon. The icos has been tested in low pressure environments inside a vacuum chamber to analyze the performance of the spectrometer and the thermal levels of components using a thermal camera. At low pressure, the thermal levels of the icos were found to increase suddenly, but plateau in acceptable temperature ranges. Ongoing experiments will continue the development of this technology. A planet’s atmosphere is full of atmospheric dust and water/ice crystals. This second study discovers if particle size and concentration can be measured from the scattering of light from lasers in order to provide useful information about the atmosphere of an observed planet. A laboratory experiment took pictures of laser beams travelling through known sized spherical particles (microspheres) and concentrations. A computer model was made to measure the change of the intensity of scattered light as the laser beam travels through these microspheres. The results are expected to find an exponential decrease of light intensity with respect to the distance travelled by the laser beam.
Christofer Cherpillod
Program: LURA
Supervisor: Michael Bazzocchi
Profile: Christofer is a 2nd Year Engineering Science Student at the University of Toronto. He was interested in this position since it provides an opportunity to work in the summer while learning about a new topic he loves. Christofer aims to research the use of shape memory alloys, a novel material to create a fully mechanical, passive system to orient solar panels to the Sun aboard a satellite.
Abstract Title: Self Orientation of a CubeSat Solar Array Using Shape Memory Alloys
Abstract Authors: Christofer Cherpillod, Michael Bazzocchi
Abstract: A passive Sun tracking system for solar array orientation onboard a CubeSat using shape memory alloys (SMAs) was designed and validated. SMAs are metals that return to a defined shape when heated. They were situated such that when the sunlight is misaligned with the solar array it heats the SMA actuator causing the solar array to move. This system increased the power output of a CubeSat’s solar array by ensuring its solar panels always point towards the Sun, maximizing their effective area. Current passive Sun tracking systems for solar array orientation do not function effectively onboard a CubeSat due to large sizes, minimal ranges of motion, or a reliance on gravity, all three of which are improved upon by this design, purpose built for CubeSats. Most current CubeSat systems employ sensors and electronic actuators to orient solar arrays, but this process uses electricity that could otherwise be used by the rest of the satellite. This means a passive approach using shape memory alloys to both sense the Sun’s position as well as actuate motion increases the total generated usable power of the solar array system. Various design concepts using shape memory alloys to passively orient a CubeSat’s solar array to the Sun were modeled then validated against the CubeSat standard before demonstrating that these designs meaningfully increase the power produced by a CubeSat’s solar array. Previous simulations show 60% greater average power output over one orbit of a satellite using a solar tracking system and similar results are expected. This technology provides a way for CubeSat manufacturers to increase power output whilst not increasing size and complexity allowing for CubeSats to take on bigger and better missions.
Connor Humphries
Program: USRA
Supervisor: Regina Lee
Profile: Connor is a 3rd year Space Engineering student continuing research in the
Nanosatellite Research Laboratory as it has a focus on Space Situational Awareness. In layman's terms the detecting, tracking, simulating, avoiding and de-orbiting of space debris or junk. Connor is hoping to become one of the world's greatest trash men by assisting in future de-orbiting missions.
Abstract Title: Interpolation to Extrapolation, Expanding the Space-Based Optical Imaging Simulator for Adaptive RSO Detection and Tracking Algorithms
Abstract Authors: Connor Humphries, Matthew Driedger, Paul Harrison, Randa Qashoa, Regina Lee
Abstract: The rapid increase of Resident Space Objects (RSOs) necessitates effective Space Situational Awareness (SSA) programs. Machine Learning (ML) methods can enhance SSA by automating RSO detection and tracking in optical images. However, generating labeled training sets for ML algorithms is tedious and prone to human error. Image simulators such as the Space Based Optical Imaging Simulator (SBOIS) developed at York University can support SSA ML algorithm development by providing training image data. This research enhances SBOIS to generate predictive image sets based on propagated observer and RSO ephemerides, enabling its use in larger simulations with active attitude control.In previous iterations, the SBOIS simulation environment was used as a passive historical interpolation tool which created image databases for RSO detection algorithms. By extending the SBOIS simulation environment into a live-feedback extrapolation tool, capable of propagating future observer and RSO positions to generate predicted images, SBOIS can be integrated into larger simulation environments. These improvements are especially useful in developing adaptive RSO detection and tracking algorithms.The standard method for tracking and imaging an RSO using a space-based SSA platform is to generate a set of ground-based pointing references to direct the observing satellite’s attitude toward the target RSO. Ground-based pointing references are sensitive to errors in the RSO’s assumed ephemeris due to factors such as orbital perturbations, anomalies, and RSO maneuvers. These errors lead to difficulties in maintaining the target RSOs within the observer’s field of view, especially in Low Earth Orbit (LEO). Using the expanded SBOIS simulation environment presented here, researchers can develop and test adaptive RSO detection and tracking algorithms to maintain target RSOs within an observing spacecraft’s field of view. These adaptive pointing references can bypass the errors inherent in ground-based pointing references and significantly improve the capabilities of on-orbit SSA platforms.Emilee Taylor Brown
Program: USRA
Supervisor: Regina LeeMishal Vellani
Program: LURA
Supervisor: Gunho SohnProfiles: Emilee is a 2nd year Chemical Engineering student at McMaster University. She joined Dr. Regina Lee’s Nanosatellite Research Lab in May of 2024 as an Undergraduate Research Assistant. She has chosen to participate in research over the summer to learn more about the field and gain experience for future opportunities. Mishal, an upper-year Earth and Atmospheric Science student specializing in Space Science at Lassonde School of Engineering is driven by a profound curiosity about the world and a passion for understanding the Why and How. Her fascination with Science and outer space fuels her belief in the power of research to drive significant advancements. With a desire to apply her expertise to enhance space exploration and improve quality of life, Mishal embodies a relentless commitment to scientific inquiry and exploration.
Abstract Title: Open-source Resident Space Object Detection Pipeline
Abstract Authors: Emilee Brown, Aiden Sanvido, Mishal Vellani, Regina Lee, Gunho Sohn
Abstract: Nearly 27,000 resident space objects (RSOs) currently populate low-Earth orbit, with numbers expected to rise significantly. This increase necessitates robust detection and tracking techniques to prevent collisions among satellites. This research introduces an open-source website designed to facilitate the detection and tracking of RSOs through user-uploaded night-sky image sequences. Current tracking methods are limited in accessibility and often require manual correction for accurate annotation. The lack of easily accessible and accurate tracking tools poses a risk to the growing number of satellites and RSOs. This study aims to provide an accessible platform for tracking RSOs and automating the annotation process to improve the quality and availability of truth data for satellite tracking algorithms. The proposed platform leverages the PFT+ (Proximity Filtering and Tracking) algorithm, which processes uploaded night-sky image sequences to determine the trajectory and physical characteristics of detected objects. Additionally, a downloadable annotation tool is provided for manual correction and refinement of the algorithm’s results. The PFT+ algorithm was tested on the Resident Space Object Near-space Astrometric Research (RSONAR) payload dataset, comprising 429 unique images across three sequences. The algorithm achieved an average precision score of 98.5%, a recall of 76.3%, and an F1 score of 86%, demonstrating high accuracy in object detection and tracking, while reducing manual annotation needs. The downloadable annotation tool further allows refining of the data through manual corrections. This open-source website streamlines the production of accurately labeled truth data, crucial for enhancing AI-based tracking algorithms and improving space situational awareness. By allowing global contributors to share novel truth data, the website reduces information bias and increases training data for existing algorithms. This democratization of truth data is essential for improving the tracking of space debris and preventing future collisions in Earth's crowded orbit.
Eric Tu
Program: LURA
Supervisor: Jinjun Shan
Profile: Eric will be going into their fourth year of Space Engineering at York University. He chose to participate in research because of his desire to create new knowledge and contribute to developing new technologies. Additionally, going into research serves as an opportunity to develop complex problem-solving skills. As opposed to schoolwork in which there is always a specified answer, research allows for the individual to carve their own path, developing a unique solution to said problem.
Abstract Title: Autonomous Drone Racing Simulator
Abstract Authors: Eric Tu, Amaldev Haridevan, Jinjun Shan
Abstract: Autonomous drones have a plethora of applications, serving as a valuable tool in several industries. They are capable of surveying areas, conducting search and rescue missions, and performing inspections and maintenance-related tasks. A factor paramount to the success of autonomous drones lies in the robustness and safety of the algorithms implemented. Autonomous drone racing serves as a method to develop these algorithms, where the goal is to have the drone traverse a course of obstacles within a short period of time. However, testing these algorithms can be very costly and create a variety of safety concerns. To eliminate these risks, the algorithms can be tested in a simulator and exposed to different scenarios before physical implementation. While simulators do exist, many run off Gazebo Classic instead of Gazebo. The end-of-life (EOL) of Gazebo Classic is projected for 2025, and Gazebo offers support to Robotic Operating System 2 (ROS2). The benefits of utilizing ROS2 lies in the efficiency, flexibility, and the architecture (multi-threading execution) which addresses the single point failure issue plagued by ROS. The objective of this project is to create a simulator to complete the task of drone racing. The simulator will be used to replicate a drone racing scenario, allowing for different algorithms to be implemented and tested. The expected result is enabling a drone to complete the course in the simulated environment. By creating a simulator for drone racing, it can subsequently be used for further research and developing innovative algorithms, striving for faster times and safer trajectories. Ultimately, this work contributes to the advancement of drone technology.
Ian Porto
Program: LURA
Supervisor: Regina Lee
Profile: Ian is a 4th year Space Engineering Student who has been researching under Professor Lee for the last year. Ian really enjoys discovering new methods of solving problems. Ian will be researching Initial Orbit Determination, which is the process of finding where an object in space is and how it is moving.
Abstract Title: Initial Orbit Determination Development
Abstract Authors: Ian Porto, Vithurshan Suthakar, Randa Qashoa, Regina Lee
Abstract: Space Situational Awareness (SSA), encompassing the tracking and prediction of Resident Space Object (RSOs) locations have become more critical than ever. Evaluating the orbital elements of an RSO from optical data requires the use of angle- only observations, where sensor accuracy becomes a limiting factor. In this study, we develop a method of Initial Orbit Determination (IOD) that provides a starting point for other methods such as Unscented Kalman Filters (UKF). By utilizing the orbital Keplerian elements obtained from the analysis of Starlink satellites and the Cascade SmallSat and Ionospheric Polar Explorer (CASSIOPE), we validated our orbital elements evaluation for the IOD and UKF implementation. Preliminary analysis shows that application of the UKF with the developed IOD presents a promising solution with an accuracy of 0.02 km RSOs commonly affected by degeneracy issues. The successful implementation of this method would significantly improve our ability to track RSOs, thereby reducing the likelihood of collisions and the creation of large debris fields in space.
Hao Zhang
Program: LURA
Supervisor: Jinjun Shan
Profile: Hao Zhang is a 4th year Computer Science student, interested in solving real world problems with technology, especially in self-driving. This is why his research focus will be on solving real-world problems with technology, and specifically on self-driving.
Abstract Title: Scalable Real-Time System for Autonomous Driving: A Case Study from ACC2024
Abstract Authors: Hao Zhang, Jinjun Shan
Abstract: One of the main challenges faced by self-driving vehicles is how to control and detect objects correctly in real time with limited computing resources. This places high demands on the system's responsiveness and the vehicle's control capabilities, and we also encountered this challenge in the American Control Conference's student self-driving competition. Our autonomous driving platform, QCar, had to correctly recognize traffic light signals and stop signs at high speeds without significant deviations. Moreover, due to the high difficulty of the competition, our team prepared several strategies for different scenarios, requiring the system to have good scalability to support rapid policy switching. To address these challenges, I utilized agile development methodology and developed a pseudo-real-time operating system based on asynchronous programming and my teammate's reinforcement learning (RL) system. This system has one central process with multiple threads specifically for IO operations, such as getting image data from on-board cameras, transmitting it to other modules, applying actions to the QCar, and interfacing to provide observations to add-on modules like YOLO-based object detection, transformer-based vision lane following, and LiDAR-based simultaneous localization and mapping. This architecture achieved real-time parallel computing with limited computational resources, ensuring high control frequency and stable observation acquisition, enabling QCar to accurately identify targets and make correct responses at high speeds. Ultimately, our solution won second place. Since the system adopts microkernel architecture principles, it also has strong scalability. Therefore, PhD candidates in the lab plan to utilize it in their research, such as developing RL methods for real-world scenarios and integrating humans in the loop in the RL training.
Luca Cherpillod
Program: LURA
Supervisor: Gunho Sohn
Abstract Title: Monocular Depth Estimation for Autonomous Drones Using Fisheye Cameras
Abstract Authors: Luca Cherpillod, Gunho Sohn
Abstract: There has been a significant increase in interest of Autonomous vehicles such as drones due to their versatility across a wide range of applications. Precise depth estimation is crucial for these drones to navigate their environment. Traditionally, techniques such as LiDAR or Visual Inertial Odometry (VIO) have been employed. However, these techniques often suffer from high computational complexity, or a high hardware demand. This research project explores the use of monocular depth estimation techniques for fisheye cameras, aiming to utilize readily available hardware with the power of machine learning to provide accurate and efficient depth perception for autonomous drones. Depth estimation using fisheye cameras pose challenges due to image distortion, variable camera parameters, and the lack of publicly available datasets. Our methodology is to adapt the existing depth estimation model, fastDepth, for fisheye cameras. Using multiple datasets, including NYU Depth v2 and JBNU_Depth360, we trained and evaluated the model for its accuracy. The NYU Depth v2 dataset provides a diverse range of indoor scenes, while JBNU_Depth360 offers indoor fisheye images with corresponding depth data, making them complementary for our needs. We evaluated the model's performance and speed on our drone hardware, namely the Starling V2 from ModalAI. Our model's performance is then validated not only through traditional AI metrics but also through practical tests involving obstacle avoidance during drone flight, demonstrating its applicability and impact in real-world scenarios. In long run, we aim to provide more efficient and reliable depth estimation, by incorporating fisheye cameras, facilitating efficient and low-cost drone navigation solutions.Marissa Myhre
Program: USRA
Supervisor: Regina Lee
Profile: Marissa is a fourth-year Space Engineering student at Lassonde. This is her second summer participating in undergraduate research. She chose to participate in research because she plans to pursue a research-based graduate program after completing her degree in Space Engineering. Marissa will be researching and developing an algorithm to determine a spacecraft's angular rate from star field images.
Abstract Title: Satellite Angular Velocity From Starfield Images
Abstract Authors: Marissa Myhre, Regina Lee
Abstract: Determining a spacecraft’s attitude is fundamental to its navigation and control, traditionally achieved using a combination of sun sensors, magnetometers, star trackers, and three-axis gyros to measure angular rates. Star trackers, known for their high accuracy in attitude determination, also have the potential to determine angular rates, a job typically handled by 3-axis gyros which are often expensive. A spacecraft's attitude can be accurately determined from a single starfield image, and using two sequential images or one long exposure image containing streaking stars, it is possible to determine the spacecraft's angular rate. Utilizing star trackers for angular rate determination presents a cost-effective alternative to 3-axis gyros for small satellite missions and provides redundancy in case of gyro failure in larger satellite missions. By determining the paths of the n brightest stars across the image through time, a statistical model can be used to estimate the occurring 3D translation or, in other words, the angular rate. The accuracy of star tracker angular rate estimations can be tested by simulating starfield images for a satellite with a defined attitude and rate. These simulated images can be run through the rate estimation algorithm to compare results. Using this testing approach the angular rate can be accurately estimated using starfield images for both stationary and streaking stars. Star trackers present a viable alternative to traditional gyros, paving the way for more efficient and reliable spacecraft attitude determination systems.
Ruella Ella Ordinaria
Program: USRA
Supervisor: John Moores
Profile: Ruella is an incoming third-year biochemistry student with a passion for understanding the molecular intricacies of life and its potential existence beyond Earth. Inspired by the work of Dr. Moores and his colleagues in the Planetary Volatiles Lab, she is exploring the impact of extraterrestrial environments on biological molecules. With the goal of pursuing graduate school, Ruella hopes to utilize her biochemistry knowledge to contribute to interdisciplinary research in the fields of astrobiology and planetary science.
Abstract Title: Mars Microbial Survival – Estimating Bioburden Reductions on Mars Spacecraft
Abstract Authors: Ruella Ordinaria, Grace Bischof, John E. Moores
Abstract: All spacecraft are subjected to sanitization protocols prior to launch to prevent biological contamination on their planetary destinations – the bioburden, the quantity of microorganisms, on the spacecraft must be limited. For missions to Mars, the Committee on Planetary Protection Report (2021) requires the exposed bioburden to be limited to 3 × 105 spores pre-launch and 5 × 105 spores in total for all surfaces. Based on the Lunar Microbial Survival (LMS) model, a first-order model that calculates the bioburden reduction of landed spacecraft on the Moon by Schuerger et al. (2019), a Mars Microbial Survival (MMS) model is developed to assess forward contamination on Mars spacecraft. This study considers the effects of the most deleterious space conditions including high vacuum, extreme temperatures, solar UV radiation, and ionizing radiation such as solar wind particles (SWPs) and high-energy particles (HEPs), on terrestrial microorganisms. The MMS model uses equations from the LMS model and data from the Mars Climate Database and JPL Horizons Systems to assess the survival of _Bacillus subtilis_ on the external surfaces of nine Mars spacecraft. Results show that the synergistic effect of temperature and vacuum dominates; with this biocidal factor alone, all spacecraft immediately begin to accumulate lethal doses (LD) > 0 on its first day of cruise for all surfaces oriented at an angle of 88.99 degrees to the Sun. For example, Phoenix collected the highest bioburden reduction during its transit to Mars while directly oriented to the sun, it accumulated a total of -1.22 x 106 logs of reduction. It is unlikely that spacecraft sent to Mars will carry any viable terrestrial microorganisms upon its landing, due to the sterilization effects of the biocidal factors present in the space environment during its transit.
-
Electrical Engineering & Computer Science
Adnan Ahmed
Program: USRA
Supervisor: Franck van BreugelHiva Karami
Program: LURA
Supervisor: Franck van Breugel
Profiles: Adnan is a 2nd-year Computer Science student with a passion for research and a drive to pursue a master's degree. Seeking to broaden his knowledge and gain valuable experience, he chose to participate in research projects. Through this endeavor, Adnan aims to develop skills that will not only enhance his academic journey but also contribute to his future pursuits in advanced studies and professional endeavors. Hiva is a second-year computer science student with an interest in theoretical computer science. She has a background in competitive programming. She chose to participate in the research because she's eager about the chance to work with experienced professors like Professor Franck van Breugel. This opportunity will help her prepare for a master's degree in computer science. Her goal is to enhance her understanding of complex computer science ideas and improve her problem-solving skills.
Abstract Title: Bug Detection in Randomized Algorithms
Abstract Authors: Hiva Karami, Adnan Ahmed, Franck van Breugel
Abstract: Testing is essential in software development as it plays an important role in ensuring the reliability and functionality of systems. Without proper testing, system failures can result in substantial costs and jeopardize user and data security. However, the presence of randomness in algorithms makes traditional testing methods challenging. This project explores the application of probabilistic model checking to identify bugs in algorithms that exhibit randomness. Currently, PRISM, developed at the University of Oxford, is the most popular tool used for probabilistic model checking and It detects bugs in a system that relies on randomness by checking properties of a model of the system. One significant challenge, known as the state space explosion problem, is that the models generated are often very large, and checking properties in these models can consume lots of time and memory. To address this, we focus on model minimization techniques. By identifying states that exhibit similar behaviors, we can reduce the size of the model and significantly improve the efficiency of property checking. Besides the minimization algorithm in PRISM, we have also implemented three known algorithms that perform the minimization step within PRISM and have measured their running time against each other as well as that of the original minimization algorithm in PRISM. Additionally, we have expanded PRISM so that anyone in the future can implement their own minimization algorithm and use it within PRISM. This work contributes to PRISM and, as such, offers practical solutions for improving the reliability of systems that rely on randomness.Aijaisarma Sabaratnasarma
Program: USRA
Supervisor: James Elder
Profile: Aijaisarma is a 4th year Computer Science student at York University. His expertise and field of interests include Computer Vision, Machine Learning and Deep Learning. He chose to participate in this research internship to gain valuable academic experience to pursue a Masters or PhD in the future. As well as to work with and network with fellow grad students and professors and learn more about Computer Vision Research and develop his engineering skills. Aijaisarma wants to make an impact in his community with his technical skills and believes pursuing research is the way he can change the world.
Abstract Title: Real-Time Deployment and Performance Optimization Strategies for Vehicle Counting
Abstract Authors: Aijaisarma Sabaratnasarma, Shreejal Trivedi, James Elder
Abstract: Object tracking is a fundamental computer vision problem that appears across many applications. One of these applications is tracking vehicles along highways and roads for gathering analytics and optimizing transportation infrastructure. The deep learning pipelines used in vehicle tracking generally consist of 3 phases: detection, tracking and counting. For real-time detection, detectors such as RTDETR and trackers such as YOLO or ByteTrack can be used alongside an AI-driven video processing toolkit to connect phases of the pipeline, in our case NVIDEA DeepStream. At the end of the pipeline when aggregating highway lane counts, clustering algorithms are traditionally used which can be computationally intensive if not implemented correctly. Thus, we approach counting optimization by converting Python code into memory and time efficient C++ code and directly binding C++ files into the DeepStream pipeline. Here we show that the frame rate for vehicle counting can be increased from approximately 700 FPS to 1000 FPS allowing us to design an edge computing model for deployment. This type of deployment consists of running the pipeline all on the NVIDEA Jetson GPU on-site at Highway 401, where the counting algorithm is executed remotely and the results of the lane counts are sent back to our lab. Our results showcase how crucial performance is when designing real-time systems and why small-scale optimizations should be considered when implementing object detection pipelines with large video datasets. We expect further optimizations can be made on an algorithmic and software level alongside our hardware optimizations. For instance, to ensure a reliable system, an OS-based virtualization deployment platform such as Docker can be used to containerize our pipeline allowing for remote reboots, which is only possible with fast performance and communication. Thus, a thorough analysis of datasets and code with rigorous benchmarking is essential for future efficient vehicle tracking models.Ailiya Jafri
Program: LURA
Supervisor: Meiying Qin
Profile: Ailiya is a first-year student in Computer Engineering at Lassonde School of Engineering. She has a keen interest in robotics and programming driven by a lifelong fascination with mechatronics. Ailiya pursued research this summer to share her passion with her peers by making this area of studies more intuitive for future students.
Abstract Title: Using Augmented Reality to Visualize Python Code Execution
Abstract Authors: Ailiya Jafri, Meiying Qin
Abstract: Although programming is gradually integrating into the primary and secondary school curriculum, it is still an unfamiliar subject to many first year university students. Debugging, which is fixing code, is especially challenging as students have difficulty thinking like a computer and imagining how their programs work. Many applications are created in order to target this weak spot in education, including websites that allow students to input Python code and view the state of the variables and values at each line of the code’s execution. There are also applications that use augmented reality (AR), which is the layering of computer graphics over the real world, to visualize the structure of the program. However, no one has developed an application which utilizes the immersive benefits of AR in order to visualize not only the structure, but also the memory of Python code. The objective of this project, then, is to develop an application that provides students with a comprehensive view of their program’s state and functionality in order to ease the process of debugging for programming newcomers. We are developing our application so that students are able to input their own Python code and navigate through its AR visualization. Our application is able to read Python code and uses the metaphor of a factory assembly line to visually demonstrate how the user’s program works. While it is still in the early phase of development, once complete it has the potential to become a powerful tool for first year programming classes. Its possible capabilities also extend to helping students develop good coding style. In the future, this application will be tested on first year classes to gather data on the student perspective in order to make improvements.
Arian Haghparast
Program: LURA
Supervisor: Manos Papagelis
Profile: Arian is entering his third year of Computer Science at York University, where he is exploring his passion for machine learning under the guidance of Dr. Papagelis. Since high school, he has been intrigued by computers and their potential to mimic human learning capabilities. He is particularly interested in reinforcement learning and its applications to real-world problems. By participating in this research, he wants to deepen his understanding of AI, contribute to innovative solutions, and prepare for a career that advances the field of artificial intelligence.
Abstract Title: Network-aware Multi-agent Reinforcement Learning for the Vehicle Navigation Problem
Abstract Authors: Arian Haghparast, Fazel Arasteh, Manos Papagelis
Abstract: Traffic congestion in urban road networks leads to slower speeds, longer trip times, increased air pollution, and driver frustration. This congestion occurs when traffic demand exceeds the available street capacity and is often worsened by incidents, road maintenance, and bad weather. Building new infrastructure is costly, so optimizing traffic flow through route planning algorithms is a more economical and sustainable solution. Popular navigation services like Google Maps and Waze use variants of the Shortest Path First (SPF) algorithm, which assumes static conditions. However, road conditions are dynamic, causing SPF to perform sub-optimally. Additionally, SPF’s greedy nature can lead to congestion by routing all vehicles along the same path. To address these limitations, we propose a network-aware multi-agent reinforcement learning (MARL) model for vehicle navigation. This model adapts to current traffic conditions by assigning a reinforcement learning (RL) agent to each road intersection. These agents act as routers, learning the optimal routing strategies over time and providing dynamic routing instructions. A vehicle, aware of its destination but not the entire route, queries the RL agent at each intersection. The agent’s response considers (i) the vehicle’s destination, (ii) current traffic conditions, and (iii) strategies learned in cooperation with neighboring RL agents. The vehicle follows these dynamic instructions until it reaches its destination. Extensive experiments on both synthetic and realistic road networks show that our MARL model can reduce average travel time by up to 17.3% compared to the SPF algorithm. This approach offers a more efficient and adaptive solution to urban traffic management, reducing congestion and improving travel times.Baran Parsai
Program: LURA
Supervisor: Michael Jenkin
Profile: Baran is a first-year computer science student at Lassonde, who joined the LURA program this summer to explore advanced programming for enhancing human-robot interaction. She will be working with Professor Jenkin at the Fields Robotics Lab on the "Emotionally Aware Avatars" project. This opportunity allows Baran to engage with cutting-edge technology and contribute to innovative research in the field of programming, aligning with her academic and professional aspirations.
Abstract Title: Emotionally Aware Avatars in Support of Human-Robot Interaction
Abstract Authors: Baran Parsai, Michael Jenkin
Abstract: Emotionally aware avatars have the potential to significantly enhance human-robot interaction by providing a more intuitive and engaging user experience. While current human robot interaction systems lack the ability to interpret and respond to human emotions effectively, this limitation can hinder their acceptance and efficacy in real-world applications. To address this gap, we developed a face recognition module designed to detect users and categorize their roles in real-time, integrating this capability into avatars for improved interaction with robotic systems. The objective of this project is to enhance the acceptability and usefulness of robotic avatars. We employed advanced facial recognition algorithms to identify and categorize users based on their roles from live video feeds. The module was trained using a comprehensive dataset of facial features, ensuring high accuracy in user detection and role categorization. We then integrated this module into a robotic avatar system, allowing the avatar to respond appropriately based on the detected user roles and their distance from the camera. The face recognition module successfully detected users and provided categories for their roles, such as administrators, operators, and visitors. When integrated into the avatar, the system adjusted its responses to match the detected user roles, resulting in a more engaging and interactive experience for users. The development of emotionally aware avatars represents a significant advancement in human-robot interactions, offering the potential to create more empathetic and user-friendly robotic systems. This research sets the stage for future developments in this field, ultimately aiming to improve the integration of robots into everyday human activities.
Behrouz Homam
Program: LURA
Supervisor: Razieh Salahandish
Abstract Title: Smart Goggles for Early Detection and Monitoring of Alzheimer's Disease through AI-Based Ocular and Cerebral Biomarkers
Abstract Authors: Behrouz Homam, Neda Salahandish, Alireza Norouzi Azad, Ariel Feygin, Arman Laly, Shahak Rozenblat, Jordan Rimon, Raha Golsorkhi, Shaylin Ziaei
Abstract: Alzheimer's disease (AD) presents a significant challenge due to the absence of a cure, making early diagnosis essential. This study aims to identify a panel of ocular and cerebral biomarkers, and their correlations for more accurate association with AD, using AI-based image and motion analysis, captured by novel wearable smart goggles. These goggles facilitate point-of-care (PoC) detection and personalized monitoring of AD. This research involves a four-phase plan: (1) Conduct a clinical case-control study to collect iris and retinal images, eye movement, and brain signal data from AD patients and healthy individuals. (2) Utilize AI-based analysis to develop deep learning models for predictive biomarkers. (3) Design and develop smart goggles for on-site capture and analysis of ocular and cerebral markers. (4) Assess and optimize the functionality and performance of the goggles in real-world settings. This research hypothesize is that non-invasive ocular biomarkers, coupled with brain signals, can effectively distinguish between AD patients and healthy individuals. The integration of these markers into a panel approach, analyzed through wearable smart goggles, is expected to significantly enhance on-site detection and monitoring capabilities. This study's findings will contribute valuable clinical data and advanced AI models to the scientific community, facilitating larger-scale diagnostic and therapeutic research. The developed smart goggles offer a groundbreaking solution for remote AD monitoring, improving diagnosis efficiency and reducing burdens on patients, caregivers, and healthcare systems. This innovation has the potential to transform current AD diagnostic, prognostic, and therapeutic practices towards more automated and personalized care, ultimately enhancing patient outcomes and healthcare delivery.Deepta Adhikary
Program: LURA
Supervisor: James Elder
Abstract Title: Markov Chain Monte Carlo Method for Generating Naturalistic Shapes
Abstract Authors: Prachurya Deepta Adhikary, James Elder
Abstract: The human eye perceives planar shapes using both local and global features. However, the extent to which local features, such as curvature, contribute to perception is currently unclear. To stimulate research in this area, we have developed a generative computational model that can, given a set of example shape contours, produce synthetic shapes with the same underlying curvature statistics as the example set. Our model generates new candidate shapes by applying isoperimetric deformations to an arbitrary starting shape and then evaluates their candidacy using a Markov Chain Monte Carlo (MCMC) method. We generate four classes of synthetic shapes, each varying in the degree to which curvature statistics are matched to the example set. We utilize MCMC to progressively match the aggregate variance, skew, and kurtosis of the synthetic shape’s curvature distribution to that of the example set. The final of these four classes matches the full marginal distribution of the example set’s curvature statistics. These synthetic shapes have proven to be valuable stimuli in studying human psychophysics and non-human primate physiology, elucidating how local curvature impacts the perception of planar shapes in humans and other primates. Furthermore, we have improved upon previous iterations of our model by introducing a methodology to generate synthetic shapes from a single example shape, thus increasing the diversity and usefulness of the synthetic stimuli. In this study, we present synthetic stimuli generated from example contours of animals (animate) and tools (inanimate). Our results demonstrate the efficacy of the present model and pave the way to understanding how the human visual system encodes shape.Greatlove Bariboloka
Program: LURA
Supervisor: Kai Zhuang
Profile: Greatlove is a 4th year Software Engineering student. They are interested in software design and development that makes user experiences smoother. As a student, they have supported many peers as a mentor, organized competitions and have acted as a teaching assistant. They chose research at Lassonde because their desired project would make the learning experience more enjoyable and memorable for first year students.
Abstract Title: Enhancing First Year Embedded Systems Learning Through Music Production
Abstract Authors: Greatlove Bariboloka, Kai Zhuang
Abstract: Computational thinking is crucial for students looking to pursue careers in programming and introductory courses such as EECS1011 exist to establish the needed knowledge. This course entails one instructor-led and one self-led project, both built on an Arduino microcontroller. The Arduino in use is bulky and doesn’t quite promote a long-term interest in embedded systems, with only a few students ever revisiting the device after the course conclusion. We identified a better microcontroller for the price point which, due to its hand-held size and superior processing power, will spark interest and encourage students to continue exploring embedded systems. This new Arduino-compatible microcontroller resides on a device with integrated sensors and actuators called the M5Core2. In the first phase of our project, we develop a MATLAB-to-M5 library since EECS1011 is a MATLAB programming course. Phase 2 involves redesigning the instructor-led course project to revolve around music creation. Incorporating music in the classroom has been shown to boost memory and creativity, and we leverage that through the development of a musical instrument using everyday electronics such as a keypad. This opens up new possibilities for the self-led project where students can turn to their newly developed skills and understanding of music to create something impactful. The libraries we develop will be open source to allow interested individuals regardless of education background also explore the M5 through MATLAB. The enhanced learning environment that we create caters to students with diverse learning styles and improves the likelihood of students continuing to learn about embedded systems, thereby furthering the UN SDG Goal 4: ensuring inclusive and equitable quality education and promoting lifelong learning opportunities for all.Hashir Jamil
Program: USRA
Supervisor: Zhen Ming (Jack) Jiang
Abstract Title: Prompt Tuning in Large Language Models Using Declarative Programming: Program Repair and Test Generation
Abstract Authors: Hashir Jamil, Yinghang Ma, Zhen Ming Jiang
Abstract: Artificial intelligence applied to software engineering tasks is a key area of research for improving developer productivity. One such software engineering task is automated program repair (APR). APR is the process of patching bugs and generating tests with minimal developer intervention. Some of the most powerful artificial intelligence tools are the foundational Large language models (LLMs), such as GPT3.5. These models offer powerful potential for code generation tasks in APR as they handle program semantics at a robust level. Previous APR tools do not work with program semantics and use other methods to work. To leverage LLMs for APR, pipelines must be built to go from code/documentation to newly generated code, which requires developers to prompt language models to return the desired results. Prompt engineering is the process of building inputs for LLMs to get repeated and consistent results. In this project, LLM prompting is tuned using the declarative programming framework, DSPy. DSPy is applied to existing APR tools that leverage LLMs. The main research question asks if prompt tuning can improve results for prompt engineering versus traditional trial and error methods found in the APR tools. The expected results are minor improvements in generated code as well as improved maintainability of the LLM pipeline. The significance of this project is the transformation of trial and error approaches for prompt engineering to a programmatic approach. This allows for the use of modules and functions to serve as abstractions that handle LLM queries. Without this paradigm, prompt engineering is reduced to hard coding of prompts and poor extensibility of a project with an LLM pipeline. This is a powerful step in ensuring long term viability of applications that leverage LLMs.Ismail Adam Knapik
Program: USRA
Supervisor: Konstantinos Derpanis
Abstract Title: Improved Digital Image Quantization via Invertible YUV-space Geometric Transforms
Abstract Authors: Ismail Knapik, Konstantinos Derpanis
Abstract: Digital images produced by consumer-grade and mobile camera ISPs are almost invariably saved in the sRGB (“standard RGB”) color gamut at 8-bit depth, in line with the capabilities of most consumer-grade displays. The bit depth of a RGB image refers to the number of bits used to “quantize” each color channel - an 8-bit image thus encoding 256 unique values per channel. 8-bit images, while generally considered “good enough” for typical media distribution, may exhibit perceptible “banding” quantization artifacts - any editing, post-processing, or lossy compression on a quantized image is likely to exacerbate this. Accordingly, the focus of this project is to develop a method to preserve more unique color tones at a given bit depth at quantization time. Here we show that there are, on average, inefficiencies in the standard quantization procedure that can be improved through geometric means. Considering an image as a 3D point cloud and constraining ourselves to a class of simple invertible linear transforms, we demonstrate a fast method to minimize bits allocated to tone ranges not present in the original image, operating in YUV space in order to exploit desirable properties of a luma-chroma orthogonal space. Intermediate results demonstrate a 16% mean increase in the number of unique tones preserved and a mean reduction in RMSE after quantization across a dataset of 153 16-bit photos. We aim to also demonstrate that our method can be integrated directly into standard lossless and lossy image compression pipelines such as the JPEG standard with relatively negligible encoding and decoding time and space overhead. The overarching goal is to demonstrate the viability of a novel class of image quantization techniques as well as their synergy with current standard pipelines, enabling higher quality digital media distribution relative to storage demands.James Fletcher
Program: USRA
Supervisor: Aijun An
Profile: James is a 4th year Computer Science student with a research focus on data mining and large language models. Through this research project, he looks forward to gaining practical experience with state-of-the-art language models.
Abstract Title: Automated Text Descriptions for Vehicle Dashboard Icons Using Large Vision-Language Models
Abstract Authors: James Fletcher, Nicholas Dehnen, Seyed Nima Tayarani Bathaie, Heidar Davoudi, Aijun An
Abstract: Vehicle dashboard icons communicate critical information to drivers, yet many drivers remain unfamiliar with their meanings. To address this, we developed a method to automatically generate text descriptions for icon images, enabling their integration into an existing voice-based virtual assistant system for drivers. We compiled a dataset of 408 vehicle dashboard icon images from 42 vehicle manuals and generated both visual and functional descriptions using three state-of-the-art large vision-language models (lvlms): gpt-4, llava, and claude 3.5. We evaluated the generated descriptions using standard metrics (bert-s, clip-s, bleu, meteor, rouge), sentence embeddings (sbert cosine similarity), and human evaluations. Gpt-4 performed the best overall, with claude 3.5 close behind, while llava performed relatively poorly. Generating accurate visual descriptions proved significantly harder than generating functional descriptions. A 5-shot learning strategy showed the most significant improvement over the zero-shot baseline. Human evaluation of 60 images showed that descriptions generated by claude 3.5 and gpt-4 were comparable to human-generated ground truth, while llava significantly lagged behind. Our key contributions include presenting a new image description dataset containing vehicle dashboard icons, demonstrating the efficacy of lvlms in generating accurate icon descriptions, and identifying sbert cosine similarity as the most reliable metric aligning with human evaluations. More broadly, our approach of automatically generating text descriptions for images using lvlms can be extended to various domains where visual symbols or icons are prevalent, such as healthcare, aviation, and industrial control systems. This research highlights the potential for using lvlms to understand and describe icons and other abstract imagery in safety-critical applications.Jaspreet Sekhon, Manpreet Sekhon, Yat Long (Cyrus) Hui
Program: LURA
Supervisor: Peter Lian
Profile: Jaspreet is a 3rd year Electrical Engineering student interested in embedded systems for biomedical applications. They chose to partake in research because they want to innovate and contribute to advancements in medical devices. Jaspreet will be researching how to create a low power cuffless blood pressure device through measuring the heart's electrical activity and blood oxygen concentration.Program: Dr. James Wu Research Internships for Undergraduate Engineering Students
Supervisor: Peter Lian
Profile: Manpreet is a 3rd year Electrical Engineering student. He chose to participate in the research because of his interest in creating low power high speed devices, specifically in biomedical applications. By participating in the research, he will be able to gain experience in this field so he can use his knowledge and apply it in the industry.Program: LURA
Supervisor: Peter Lian
Profile: Cyrus is a 2nd-year Computer Science student passionate about applying machine learning to solve real-world problems. Cyrus’s research interest lies in biomedical signal analysis and market simulations related to game theory. He believes real-time monitoring of COVID-19 can lead to quicker responses and more effective containment measures to prevent a pandemic outbreak. Therefore, he hopes to bring a positive impact through this research opportunity.
Abstract Title: Non-Invasive Blood Pressure Monitoring: The Future of Cuffless Technology
Abstract Authors: Jaspreet Sekhon, Manpreet Sekhon, Yat Long Hui, Peter Lian
Abstract: Hypertension affects around 1.13 billion people worldwide and is a huge contributor to the deterioration of cardiovascular health. Traditional methods of blood pressure monitoring, which rely mainly on inflatable cuffs, constitute serious challenges due to their uncomfortable nature and difficulty of use. This can hinder the ability for continuous monitoring and patient compliance which leads to insufficient control of hypertension. To solve these problems, we have designed, and programmed a cuffless blood pressure monitor prototype. The prototype includes a custom-made printed circuit board which integrates an ECG Front End IC and a Pulse Oximeter. The ECG Front End IC allows for electrodes to be connected, the ECG signals are then amplified and converted to digital signals. Along with this, the Pulse Oximeter gets blood oxygen concentration from the finger and sends the data via an I2C line to the microcontroller. The ECG and Pulse Oximeter data is then sent from a microcontroller via a Bluetooth signal to a desktop application which employs an algorithm that calculates and analyzes Pulse Arrival Time to get a blood pressure reading. Our design offers accurate readings of blood pressure due to digital signal processing techniques used in the firmware and hardware which eliminate majority of the noise. Furthermore, the careful selection of the components also allow our design to be ultra low power. This approach ensures accurate and trustworthy measurements for blood pressure while also improving patient compliance and comfort, opening the door to a new era of controlling hypertension and improving overall cardiovascular health.
Julian Forsyth
Program: LURA
Supervisor: Konstantinos Derpanis
Profile: Julian just completed his 4th year in Computer Science at York. He is passionate about exploring the intersection of mathematics, computation, and the natural world. Working in the Computational Vision and Imaging Lab gives the opportunity to grapple with the most interesting problems at the edge of AI and vision.
Abstract Title: Understanding and Controlling Deep Vision Networks with Sparse Autoencoders
Abstract Authors: Julian Forsyth, Matt Kowal, Konstantinos Derpanis
Abstract: As deep networks become the standard for many computer vision tasks, the need for understanding and controlling the representations of such models is becoming increasingly important for addressing ethical, legal, and scientific concerns. One of the main challenges in interpreting neural networks is a phenomenon called _polysemanticity_, whereby single neurons appear to be responsible for numerous behaviours. Polysemanticity makes it difficult to identify and isolate discrete human-understandable concepts active in a network’s behaviour. One solution to this problem is a _sparse autoencoder_, a module which can be applied to an already trained network that converts the model’s representation into a higher dimensional one that activates _sparsely_. In theory, the sparse autoencoder can disentangle the network into neurons which are _monosemantic_, each encoding distinct and separate concepts. From here, the network can not only be interpreted, but also tuned in order to modify downstream behaviour. Recently, researchers have found success applying sparse autoencoders to large language models, identifying a large number of human-understandable concepts. While sparse autoencoders have been explored in the language space, little work has been done in the computer vision realm. The goal of this project is to apply sparse autoencoders to state-of-the-art computer vision models for the purpose of identifying human-interpretable visual concepts. Visual concepts range from simple ones such as textures and shapes to more sophisticated concepts such as those pertaining to geographic landmarks or cultural activities. The results of this work can have great importance in uncovering and understanding the existence of bias in vision networks, and mitigating bias with greater success than has been previously achieved.
Karolina Tchilinguirova
Program: LURA
Supervisor: Alvine Boaye Belle
Profile: Karolina, a second-year Computer Science student, is interested in research as it will enhance her learning beyond the classroom. She is eager to gain hands-on experience to contribute to her professional development. She values the opportunity to collaborate with experts in the field, benefiting from their guidance and insights. This experience promises to provide her with a well-rounded perspective, enriching her academic journey and preparing her for future career opportunities.
Abstract Title: Gender and Racial Inequity in Citation Practices in Software Engineering
Abstract Authors: Karolina Tchilinguirova, Alvine Belle
Abstract: The number of citations of scientific articles significantly impacts recommendations for funding allocations, recruitment decisions, promotions, and awards. Recent studies in various scientific fields, such as physics and neuroscience, have found that researchers from certain socio-cultural groups, like women and racialized people, are cited less frequently than their counterparts from dominant groups. This disparity often results from unconscious citation biases in reference lists, leading to unfairness and inaccuracy in assessing the impact of articles. These biases can cause significant disparities in promotion, retention, grant funding, awards, collaborative opportunities, and publications. In this project, we present, to the best of our knowledge, the first study to analyze citation practices in software engineering literature. We hypothesize that similar citation biases observed in other fields will also be present in software engineering. Our methodology involved collecting papers from the top 100 software engineering journals over the past 20 years. Using gender and race predictive software, we assigned gender and race labels to authors based on first and last names. We then compared the proportion of authors from various racial and gender groups to the proportion of those groups referenced in the literature. Our results indicate that efforts are still needed to achieve fairness in software engineering citation practices. The proportion of women and racialized groups in the literature is significantly less than that of the dominant group. We also found that due to the smaller proportions of these groups, their citation rates exhibit much greater variability and are less consistent than those of the dominant groups. One potential solution to address these disparities is the inclusion of citation diversity statements in manuscripts submitted for publication in computing and software engineering journals and conferences.
Katherine Ling
Program: USRA
Supervisor: Jarek Szlichta
Profile: Katherine is a fourth-year Computer Science student. She is engaging in research to deepen her understanding of artificial intelligence and contribute to developing more transparent AI systems. She will study methods to explain answers produced by large language models.
Abstract Title: COuRAGE: COncepts Retrieval-Augmented LLM Explanations
Abstract Authors: Katherine Ling, Parke Godfrey, Lukasz Golab, Joel Roserth, Jarek Szlichta
Abstract: Large language models (LLMs) have seen significant growth in both popularity and capability. As LLMs integrate into critical domains, providing verifiable explanations is crucial in building trust, especially when users are presented with a ranked list of answers. The lack of transparency in how LLMs prioritize different criteria (concepts) during their responses leaves users unaware of how concepts influence ranking logic. Additionally, LLMs are prone to generating incorrect or nonsensical answers (hallucinations), further eroding user trust. One approach to reducing hallucinations in LLMs is retrieval-augmented generation (RAG). This popular prompting technique augments the LLM's knowledge with external sources supplied directly into the LLM's input context (prompt). Previous work like RAGE has provided high-level explanations for LLMs enhanced with RAG, focusing on the origin of LLM answers concerning external sources. However, a key gap remains: understanding how LLMs prioritize different concepts within a prompt to generate ranked answers. This project proposes COuRAGE, a tool building on RAGE, to explain how considering different concepts within the prompt impacts an LLM's answer ranking. We hypothesize that by perturbing the user's question to the LLM with concepts, we can determine their significance in the LLM's explanations. COuRAGE takes a user's question as input and retrieves a set of relevant sources. Then, we identify a set of concepts using methods such as directly asking the LLM, asking a different LLM, or extracting from chain-of-thoughts. The same is done to retrieve a set of potential answers. We use saliency methods like SHAP to find the importance of concepts and generate counterfactual explanations by identifying the minimal set of concepts that change an answer's ranking when removed from the prompt. This project aims to improve the transparency and reliability of LLMs by revealing how different concepts impact answer rankings, thereby contributing to more dependable AI technologies.
Laily Ajellu
Program: LURA
Supervisor: Jeff Edmonds
Profile: Laily is passionate about crafting ChatGPT to give more specific answers. She is building Fine-Tuned models based on the latest versions of ChatGPT. This involves training the models on expert-picked bodies of text to create custom document flows. Cubatorin gives quality advice to startups. To do this, Laily has applied Natural Language Processing (NLP), a subfield of Artificial Intelligence which processes large texts. She has then fine-tuned the AI's responses so that it uses expert-fed data. Cubatorin is updated multiple times a day with this data. The data is provided by Venture Capitalists and Startup Advisors (rather than the average Joe on the internet), making it 5% more actionable.
Abstract Title: Cubatorin - The Investor & Entrepreneur Sidekick
Abstract Authors: Laily Ajellu, Jeff Edmonds
Abstract: ChatGPT's responses are often too generalized. Cubatorin provides more specific answers tailored to the field of startup investing. There are a lot of repetitive questions and processes that investors and entrepreneurs need to discuss before closing a deal. Cubatorin serves as a business incubator for startups. It offers detailed answers and a structured workflow to streamline these interactions and to generate a business proposal document. The platform also facilitates sharing the business proposal between entrepreneurs and interested investors, and includes a chat interface for investors to confirm or deny proposals. Cubatorin makes its answers more specific by fine-tuning an OpenAI chatbot. The incubator team at York University gradually curates the expert data for the training, as new techniques are discovered in the field. This data is given more importance than the training data sourced for the base model of ChatGPT, which is originally formed from general information on the web. The base ChatGPT model provides an accuracy of 50%. Cubatorin aims to improve this accuracy by at least 15%. To achieve this, a minimum of 100 expert articles need to be uploaded. After each upload, investors preview the updated chatbot and rate the improvement of its responses. If the response is deemed better, the new data is retained for further training. Cubatorin's team has collaborated with the incubator team at York university, which is composed of investors. These investors currently spend about 7 hours daily on research and guiding entrepreneurs. Cubatorin reduces this burden by approximately 4 hours, assisting entrepreneurs in developing business proposals. This allows investors to allocate more time to reviewing and providing feedback on these proposals, enhancing efficiency and productivity.
Liang Mei
Program: LURA
Supervisor: Meiying QinSleimon Naimi
Program: USRA
Supervisor: Meiying QinAbstract Title: Analyzing The Effects Of Simulation Games On First Year Transition Difficulties
Abstract Authors: Liang Mei, Sleimon Naimi, Akshay Tadi, Phillip Kim, Vrushank Vaghani, Meiying Qin
Abstract: The objective of this project is to help ease the transition for first-year undergraduate students by developing an educational simulation game. This initiative targets students from diverse backgrounds, including mature students, international students, and those transitioning directly from high school, who often face significant stress and uncertainty in adapting to university life. Traditional orientation programs, while beneficial, have limitations, such as a lack of interactions and reliance on passive learning methods like slideshow presentations. Our contribution focuses on creating an engaging, interactive, innovative game to complement the York University Markham campus orientation. This game addresses the shortcomings of conventional orientations by offering a fun, experiential learning environment without time restrictions, allowing students to progress at their own pace and access the material from anywhere. The simulation game enhances the orientation experience by making it more interactive and engaging, potentially leading to better information retention and smoother adjustment to university life. The game’s design focuses on critical soft skills of university life, including time management, academic integrity, effective communication, critical thinking and personal wellness, presented through a virtual simulation environment. To evaluate the effectiveness of our game, we will compare in-game performance metrics with faculty surveys on student habits and academic performance data. Our study aims to demonstrate that incorporating this educational game into the orientation process significantly improves the transition experience for first-year students, contributing to the United Nations Sustainable Development Goal of ensuring quality education for all.Lucas Woolner
Program: LURA
Supervisor: Yves Lesperance
Profile: Lucas is a 4th year Computer Science student who will enter the Master's in Computer Science program in the fall at York University. His research interests are AI and algorithmic theory. His long-term goal is to pursue a career in research, and participating in the LURA program is a step in this direction.
Abstract Title: Abstractions of Situation Calculus Synchronous Game Structures
Abstract Authors: Lucas Woolner, Yves Lesperance
Abstract: In many applications of artificial intelligence, automated reasoning must be performed in multi-agent systems (mas), some of which require agents to act concurrently. These applications can be modelled as situation calculus synchronous game structures (scsgs) in which changes to the system are caused by all agents choosing and performing a move synchronously at each moment in time. In this context, it is often necessary for agents to plan toward individual or cooperative goals. Automated planning can become complex, so abstraction is used to improve the efficiency of this type of strategic reasoning. Using an abstraction framework proposed by lespérance, de giacomo, rostamigiv and khan in a recent paper, in this project we show how abstraction can be used in several practical contexts including manufacturing, industrial mas applications and logistics. This is done via the development of contextual scsgs models at various levels of abstraction, and mappings between these levels which show how to refine abstract strategies into more detailed strategies. In a similar manner, we explore contexts under which the constraints necessary for this type of strategy refinement to be performed break down, and find techniques to change system models in order to meet these constraints. Finally, we propose variations on the framework to employ different levels of concurrency and observability at different levels of abstraction (for example a concurrent, fully-observable abstract level mapped onto a concrete implementation which is a turn-based game under partial observability) and demonstrate both the theoretical problems in these extensions and a number of examples of their use. The latter contribution is useful for handling conflicts which occur as a result of agents acting at the same time. In total, this work demonstrates the applicability of the theoretical framework, and provides useful examples of, and techniques for, its use in different problem domains.
Michael David
Program: USRA
Supervisor: Eric Rupert
Profile: Michael is a 3rd year Computer Science student working with Dr. Eric Ruppert through the USRA program on lock-free data structures. He chose to participate in research given his passion for computer science and mathematics along with the drive to challenge himself to contribute meaningful progress to the field.
Abstract Title: The Improvement of Concurrent Lock-Free Deques
Abstract Authors: Michael David, Eric Ruppert
Abstract: As we reach the physical limit of how small a single processor can get and how fast it can perform, more importance is placed on concurrency, given the ubiquitous nature of multi-core processor architectures. Many modern computer systems are developed to support running operations in parallel over multiple processes, and what aids them are concurrent data structures in shared memory. If multiple processes want to modify the same data simultaneously, typically only one will succeed and others will fail and have to retry, with the possibility of multiple failures. This research focuses on implementing a concurrent double-ended queue which is a shared memory data structure that stores a list of elements. Processes can insert or remove elements at both ends. Useful special cases are stacks and standard queues where insertions and removals happen only at one end. In earlier implementations of concurrent double-ended queues, the number of computational steps would be linear in the number of processes due to a multitude of retries to perform modifications. Recently, Shalom Asbell and Eric Ruppert published the first implementation of a concurrent double-ended queue that guarantees every process completes its operations and the number of computational steps is polylogarithmic. This research project covers further optimization of this implementation so that the number of computational steps depends purely on the number of processes in the system, with no dependence on the queue size and thus has no slowdown for a large number of elements. We use a new internal representation of the list of elements, which is broken up into smaller chunks so that updates can be made locally on these chunks, a key factor that provides optimization for a more efficient data structure.
Mohammad Hosseini
Program: LURA
Supervisor: James Elder
Profile: Mohammad is studying Computer Science at Lassonde. With a strong foundation in mathematics, software development, and AI/ML, he has gained experience as a Software Developer at EAIGLE. His passion for computer vision and research drives his enthusiasm to contribute to and further his learning in the LURA Research Program. Mohammad is eager to leverage his skills and knowledge to make meaningful contributions to innovative research projects.
Abstract Title: Ground Plane Projection for Robust Multi-Camera Vehicle Tracking at Traffic Intersections
Abstract Authors: Mohammad Hosseini, James Elder
Abstract: Video-based analysis of traffic at intersections requires tracking the movement of objects across multiple cameras, as single-camera systems face significant challenges due to occlusions and limited viewpoints. This research explores a method for Multi-Camera Multi-Object Tracking (MCMT) by focusing on single-camera tracking and subsequent association of these tracks across multiple cameras. Our approach leverages the calibrated nature of the cameras to project the estimated mass center of vehicles onto the ground plane, facilitating more accurate association of single-camera tracks. We aim to explore different methods for approximating the projection of the mass center of vehicles on the ground in the image plane. For this task we will investigate methods using segmentation masks, bounding boxes, and monocular depth estimation. We also explore different methods of projecting the vehicles’ mass centers into a common ground plane. This unified ground plane serves as a reference frame, enabling the fusion of single-camera tracking data from different perspectives. We will assess the accuracy and robustness of these methods in real-world traffic scenarios and evaluate techniques for associating the projected tracks from all cameras onto the ground plane. Our hypothesis is that using object segmentation and monocular depth estimation, we can achieve a good estimation of the vehicle mass center in the image plane, which will simplify the association process, making it more robust and accurate. This method addresses the limitations of feature-based tracking, which can be impractical due to the similarity in car models and colors, as well as frequent occlusions in traffic scenes. By focusing on ground plane projections, we aim to develop a more reliable system for multi-camera tracking, ultimately enhancing the precision and effectiveness of vehicle monitoring at intersections.
Mustafa Yildirim
Program: LURA
Supervisor: Hina Tabassum
Profile: Mustafa is a 4th-year Computer Engineering student at Lassonde. He is a hardworking student who excels under pressure and meets tight deadlines, as reflected in his academic performance. Mustafa has demonstrated strong teamwork and leadership by actively participating in and leading various group projects. These experiences have prepared him to contribute effectively to team efforts. He is very interested in machine learning and decided to join this research to learn more and understand new ideas. Mustafa believes this experience will greatly benefit him if he decides to pursue a master’s degree in the future.
Abstract Title: Enhancing Data Privacy and Model Performance with Federated Learning in WiFi Sensing Applications
Abstract Authors: Mustafa Yildirim, Hina Tabassum
Abstract: Federated Learning (FL) is a transformative machine learning approach that enables clients to collaboratively train a shared model while keeping their data localized. This enhanced privacy and security is a key benefit of FL. However, there is a need to evaluate the effectiveness of FL in specific applications, such as WiFi sensing, to assess its practical implementation and deployment. WiFi sensing utilizes channel state information to offer a non-intrusive, device-free method for human activity monitoring. This is crucial for smart homes, healthcare, and security applications. Traditional approaches, however, often face challenges with data privacy and centralized data processing, making FL an attractive alternative. By using FL, client devices can not only perform training in parallel at the edge instead of sequentially at a central server, but can also collaboratively learn and share generalizable location-independent traits about physical actions being monitored. In this approach, users train a local model and transmit their local parameters to a central server. To ensure the privacy of the model at this stage, differential privacy can be incorporated to make the local parameters private as well. This research focuses on comparing the effectiveness and efficiency of FL with traditional Neural Networks (NN) in WiFi sensing applications. The hypothesis is that FL will provide comparable performance with enhanced privacy. The study implements both FL and a standard LSTM-based NN model on the UTHAR dataset. The aim is to evaluate FL's ability to achieve high prediction accuracy across various locations, even with limited data from new environments. The decentralized nature of FL offers significant advantages in privacy preservation and data security, making it a viable option for applications where these factors are critical.
Oluwamayowa Isaac Ibidun
Program: LURA
Supervisor: Kiemute Oyibo
Profile: Isaac is a 3rd year Computer Science student with a passion for using technology to create innovative solutions that make a meaningful impact. He is excited to participate in this research project to explore how game-based learning can enhance student engagement and comprehension. In his free time, he enjoys watching movies, playing piano and working on personal projects.
Abstract Title: Designing and Implementing Mnemonics-Based Serious Games to Improve Long-Term Memory
Abstract Authors: Oluwamayowa Ibidun, Kingson Fung, Sarah Ejaz, Manmeet Walia, Marco Lopez, Man Chau Ng, Kiemute Oyibo
Abstract: University students often face significant challenges with content retention in memory-intensive courses. Addressing this issue, this research explores the integration of mnemonic devices and serious games to enhance learning and retention. Serious games are games designed for purposes beyond mere entertainment. They are often used in educational settings and have demonstrated the potential to increase engagement by making learning more fun. Simultaneously, mnemonic devices such as acronyms, acrostics, and the method of loci, have been widely recognized for their effectiveness in improving long-term memory. This research involved two systematic reviews to analyze existing literature on mnemonics and serious games comprehensively. The findings indicate that while mnemonics and serious games independently support learning, their combined application has not been extensively explored. This gap in the literature prompted us to design and implement 2 serious games using the Unity game engine. The overall purpose of this research is to evaluate the effectiveness of mnemonic-based serious games in aiding knowledge retention. In our research, we considered the importance of retrieval, review, and reflection in the learning process. Retrieval practice reinforces memory through recall, review consolidates learning through regular revision, and reflection enhances understanding by critically evaluating what has been learned. By incorporating these elements, the developed mnemonic-based serious games aim to provide a holistic and effective learning experience. This research aligns with the United Nations Sustainable Development Goal 4 (Quality Education) by promoting inclusive and equitable quality education and lifelong learning opportunities for all. Through future empirical studies, we aim to assess the impact of our developed games on students' learning outcomes in memory-intensive courses. This evaluation will provide valuable insights into the potential of integrating mnemonics and serious games as a novel educational strategy.
Pranav Gupta
Program: USRA
Supervisor: Manos Papagelis
Profile: Pranav is a senior student at York University, majoring in Physics with Honors and Computer Science, and a recipient of the Fulbright Scholarship. His journey is fueled by a relentless curiosity, a deep-seated passion for Artificial General Intelligence, and a commitment to establishing a significant presence in the research community. Pranav is drawn to research because it allows him to explore and solve complex, open-ended problems while enjoying the autonomy and ownership that this field offers.
Abstract Title: Enhancing Trajectory-User Linking Using Higher-Order Representations and Graph Convolutional Networks
Abstract Authors: Pranav Gupta, Manos Papagelis
Abstract: Trajectory-user linking (TUL) is a significant challenge in trajectory classification, essential for applications such as personalized recommendations, identity verification, and urban planning. Traditional methods often grapple with issues of data sparsity, quality, and imbalance. To address these, we propose an extension to the TULHOR model (Trajectory-User Linking using Higher-order Mobility Flow Representations) by integrating a Graph Convolutional Network (GCN) layer. The original TULHOR model leverages higher-order representations inspired by BERT to effectively link anonymous trajectories to users, but it falls short in capturing complex spatial relationships. GCNs, with their ability to operate directly on graph structures, are adept at learning spatial dependencies, making them ideal for enhancing the TULHOR model. Our enhanced TULHOR model, incorporating a GCN layer, is evaluated across seven diverse datasets: Drive, Porto, Rome, Geolife, NYC-Taxi, and Foursquare for Tokyo and New York. These datasets provide a robust evaluation framework, encompassing a range of trajectory data from taxi routes to GPS-based activity records. Our experiments demonstrate that the GCN-enhanced TULHOR model outperforms the original TULHOR model, particularly in scenarios where side information such as timestamps and points of interest are excluded. Accuracy improvements of 1-2% were observed across all datasets, with similar gains in precision, recall, and F1-score. The contributions of this paper are threefold: first, we introduce a GCN layer into the TULHOR model, significantly enhancing its spatial learning capabilities; second, we provide a comprehensive evaluation across multiple datasets, showcasing the model's robustness and applicability; third, we present detailed analyses of the GCN layer's impact on model performance, offering valuable insights for future research. Our findings highlight the potential of GCNs to advance the state-of-the-art in trajectory-user linking, providing a more effective tool for spatiotemporal data analysis.
Rafael Dolores
Program: LURA
Supervisor: Jarek Szlichta
Profile: Rafael is a fourth-year Software Engineering student passionate about Data Science and Machine Learning. Having spent one year as a Data Science/Engineering intern at Sanofi Pasteur, he decided to further explore this field through research and combine his industry knowledge with academic insights that he seeks to gain from the Data & AI lab. He plans to leverage this experience to pursue a master’s degree in computer science.
Abstract Title: Web-Based Automatic Data Systems Tuning Using Deep Learning
Abstract Authors: Rafael Dolores, Alexander Bianchi, Andrew Chai, Vincent Corvinelli, Calisto Zuzarte, Jarek Szlichta, Parke Godfrey
Abstract: Achieving optimal performance in databases like IBM's DB2 involves adjusting various interconnected configuration parameters known as "knobs". These knobs control system functions including accessible memory (e.g., sort-heap, buffer-pool), parallel processing settings, and optimization levels applied. Tuning these knobs is complex and error-prone, even for experts, due to the numerous configurable options. A promising recent solution, Db2une, is an automatic query-aware tuning system that has been proposed to enhance query runtimes while conserving resources. Db2une uses a machine learning pipeline to process query execution plans (QEPs) and convert them into low-dimensional vector representations (embeddings). These embeddings are fed into a deep reinforcement learning model, where an actor component suggests knob configurations and a critic component evaluates their performance, eventually acquiring optimal knob configurations. The current way of using Db2une, through a command-line that requires multiple commands for single use-cases, is limited in terms of scalability and practicality. This necessitates for the development of a web-interface that will showcase the full novelty of Db2une. A web-interface allows for a more intuitive and user-friendly experience where users can manage and adjust database configuration with ease. The main features of the present work includes model training, knob recommendations, and visual representations of the tuning process. Users have the flexibility to train different models under different settings to modify the database according to their own needs. The usage of these models for knob recommendation generates a display of comparative results which highlights the differences in runtime and resource consumption in terms of pages allocated. Moreover, it allows seamless integration with any existing Db2 databases with a valid schema and credentials. This web-interface is ought to be used primarily as an internal tool by IBM researchers and engineers. Beyond the initial scope, this tool could benefit database admins and researchers worldwide by improving database performance significantly.
Saba Yazdani
Program: LURA
Supervisor: Shahin Kamali
Profile: Saba is a third-year computer science student with a keen interest in research methodologies. She views participation in research as a valuable opportunity to deepen her understanding of complex problems and enhance her skills in algorithmic analysis.
Abstract Title: Graph Burning of Bounded Treelength Graphs: A Model for Information Distribution in Social Networks
Abstract Authors: Saba Yazdani, Shahin Kamali
Abstract: Graph burning is a deterministic, discrete-time process that can be used as a model for information dissemination in social networks. Each graph has a burning number, which quantitatively represents how quickly social influence, such as gossip or pieces of fake news, spreads through the graph. The faster a graph burns, the more vulnerable it is. The objective is to quantify this vulnerability by finding solutions that minimize the number of burning rounds. The graph burning problem is known to be NP-hard; meaning that finding an optimal solution within a reasonable time is unlikely. The problem stays NP-hard for special graph families such as trees, discrete paths, etc. Therefore, we aim to find a close-to-optimal solution that approximates the optimal solution in a feasible time. In particular, we want to bound the approximation factor that quantifies how close the approximated solution is to the optimal solution. Graph attributes such as width and depth parameters have previously been exploited to derive algorithms with good approximation factors. In this project, we study the graph burning problem for graphs of small treelength, including many natural graph families such as interval and chordal graphs. The current best approximation factor for these graphs is 2. We aim to improve this approximation factor to 1+ϵ for any small ϵ (a polynomial-time approximation scheme (PTAS)) via alternative algorithmic techniques. The problem could be challenging because the graph burning problem is a relatively new topic in graph theory. We expect that our algorithms find applications in real-world scenarios where graphs of bounded treelength model agents' physical (geometric) positions, e.g., in evaluating how fast a contagious disease may spread in a city.
Shaun Francis
Program: LURA
Supervisor: Ali Sadeghi-Naini
Profile: Shaun is a 4thyear computer science student at the University of Ottawa who wishes to continue pursuing Machine Learning in a master's program. Shaun will be researching how AI can be used to assist with the tracking of tumor cells in microscopy image series.
Abstract Title: Automated Cell Tracking in Microscopy Images Using Machine Learning Techniques
Abstract Authors: Shaun Francis, Maryam Parsian, Ali Sadeghi-Naini
Abstract: In microscopy, cells are often clustered closely together. Moreover, they often have similar shapes and properties, which made their tracking a time-consuming and difficult task. Despite this, understanding how cells move and change in response to certain medications and treatments is vital to the progression and development of the biomedical field. With the development of modern technologies, especially those in machine learning, the burden of manual cell tracking on medical research can be lessened. Computer vision tools can be used to identify cells in sequences of images. Various other computing practices can additionally re-identify cells to track movement and even changes in cell size, shape, and mitosis lineage. In this project, we have adapted modern computing tools including machine learning frameworks and computer vision libraries (YOLOv8 and OpenCV) to train custom models with the goal of identifying and tracking individual cells within various microscopy time lapses. This was achieved through partitioning a dataset of microscopy time-lapses acquired in our lab into the training, validation, and test sets to develop, train and fine-tune a custom machine learning model. Using this information, we were able to ascertain various other cell properties such as the speed at which a cell moved and quantitative displacement information. This yields several benefits and great significance to the biomedical field, namely in a reduction of reliance on manual tracking which is prone to human error, and instead providing a more efficient and accurate method of performing cell analysis. Moreover, by utilizing these tools, the biomedical field could reduce the amount of time spent manually identifying and tracking cells and spend more time engaging with the research that matters when it comes to biomedical innovation.
Sumedha
Program: LURA
Supervisor: Shahin Kamali
Profile: Sumedha is a 3rd year Computer Science student who has found a love for computer science through the theories and analyses that lie behind it. LURA is a great opportunity for Sumedha to further explore her love for CS, especially theoretical CS through her research project, which will hopefully help shape the decisions about her future.
Abstract Title: Telephone Broadcasting in Cactus Graphs
Abstract Authors: Sumedha, Seyed Mohammed, Shahin Kamali
Abstract: Information dissemination in graphs is a critical area of research, with significant implications for understanding network dynamics across various domains. One prominent example is the study of social network vulnerability to misinformation spread, which can be modelled by analyzing message broadcast speeds within the network. Telephone broadcasting serves as an abstract model for information dissemination in networks/graphs. In this paradigm, a message originates from a single node in the network, called the originator, and propagates to all others through synchronous rounds. During each round, an informed vertex transmits information to at most one of its uninformed neighbors. Telephone broadcasting has received a lot of attention in the past decades, and while polynomial algorithms exist for broadcasting in simple graph structures like trees, the problem's complexity increases substantially (technically “NP-Hard”) for more intricate graphs (e.g., graphs of bounded “treewidth”). This project focuses on cactus graphs, a unique family of connected graphs where each edge belongs to at most one cycle. Our primary objective is to elucidate the complexity of the broadcasting problem in cactus graphs. We aim to either develop a polynomial algorithm that generates optimal broadcasting schedules for any given cactus graph, or prove that no such algorithm exists (assuming “P ≠ NP”). The outcomes of this research will contribute to the broader understanding of information dissemination in networks and may have practical applications in fields such as communication systems, epidemiology, and social network analysis. By exploring the boundaries between polynomial-time solvability and NP-hardness in this specific graph family, we hope to shed light on the fundamental nature of information propagation in structured networks.
Takhmina Iliiasova
Program: LURA
Supervisor: Andrew Eckford
Profile: Takhmina is a 3rd year Computer Science student at York. She chose to participate in the research to gain experience and skills that would further help her pursue graduate studies.
Abstract Title: Exploring Logistic Growth Dynamics through the Kelly Betting Strategy: Insights from the "Game of Fitness" Simulation
Abstract Authors: Takhmina Iliiasova, Fatih Gulec, Andrew Eckford
Abstract: Understanding how populations grow and adapt to changing environments is a fundamental question in both biology and finance. The logistic growth model describes a pattern where the population expands rapidly at first, then slows down as it approaches a carrying capacity due to limited resources, resulting in a sigmoidal curve. Similar to population growth, we can model wealth growth using the Kelly betting strategy, also known as the Kelly Criterion, an investment formula used to determine the optimal bet size at each opportunity in a series of bets, leading to exponential growth of wealth over time. Despite extensive research on logistic growth, the connection between growth patterns and betting strategies remains unexplored. The objective of this project is to empirically test the hypothesis that logistic growth phenomena can be explained through the Kelly betting strategy. To investigate this, we are developing a simulation called the "Game of Fitness" inspired by Conway’s Game of Life. In the simulation, organisms reproduce at each time step, adding new organisms into the surrounding grids. These organisms have various phenotypes that are fitted to different environments. The environment changes randomly each time step, and the fitness of the population — its ability to survive and reproduce — is determined by the match of these phenotypes to the environment. We expect that the results will indicate that the Kelly betting strategy can successfully model logistic growth dynamics. Our findings are anticipated to provide new insights into the principles underlying growth processes, with implications for both biological and financial sciences.
Uktu Egemen Umut
Program: LURA
Supervisor: Konstantinos Derpanis
Profile: Utku is a second-year Computer Science student at the University of Toronto, collaborating with Professor Derpanis on a Computer Vision project. As an aspiring research scientist passionate about making significant contributions to Computer Science, Utku applied for the LURA award. This award has provided him with the unique opportunity to work alongside top researchers in Computer Vision at the Lassonde School of Engineering.
Abstract Title: A Dataset for Improving Human Pose Estimation with Rich Environmental Context
Abstract Authors: Utku Egemen Umut, Konstantinos Derpanis
Abstract: Human motion is a complex and rich phenomenon found in many types of video content, including sports events and everyday activities. Modeling human poses from visual data is a crucial area of research in computer vision and computer graphics. Many approaches to human pose estimation focus solely on the articulation of limbs of the target person, often overlooking the valuable contextual information provided by the environment, such as interactions with other agents. Moreover, existing datasets emphasize such limb articulation without capturing the broader context of these movements, including interactions with other agents and objects. The competitive chase tag is a notable example of a sport that exemplifies rich human-environment and human-human interactions. Participants navigate intricate arenas in this sport, engaging in dynamic and strategic movements to evade or tag their opponents. This setting offers a wealth of data on how individuals interact with their physical surroundings and other agents. However, a significant challenge arises from the fact that data for competitive chase tag is primarily available as video footage in a televised format. This format poses difficulties for direct analysis due to varying camera angles, occlusions, and the need for more structured annotations. These challenges necessitate techniques to extract meaningful information from such unstructured data. This project aims to extract readily usable information about the human poses of both participants and the environment (arena). By doing so, we strive to enhance the understanding and analysis of complex human motions in dynamic environments. This approach will significantly contribute to developing more accurate and context-aware pose estimation models, ultimately improving the ability to interpret and analyze human movements in various applications.
Vansh Bhasin
Program: LURA
Supervisor: Hina Tabassum
Profile: Vansh Bhasin is a first-year Computer Science student driven by a passion for exploring new domains in the industry and creating positive change through research. His primary motivation stems from the desire to understand AI and machine learning's impact on future generations and to contribute meaningfully to these fields.
Abstract Title: Deep Learning for Electromagnetic Exposure Analysis, Estimation, and Prediction
Abstract Authors: Vansh Bhasin, Hina Tabassum
Abstract: The upcoming decade will see ultra-high-speed and low-latency wireless network powered by higher transmission frequencies (e.g., millimeter waves) and ultra-dense deployment of base stations (BSs) equipped with a massive number of radiating elements. The rapid deployment of 5G networks globally has raised significant concerns regarding the escalation of Electromagnetic Field (EMF) exposure levels. This research develops a framework to generate EMF (measured in terms of power density) datasets in urban areas of Toronto considering ray tracing and topographical factors, including deflections from buildings. To achieve this, we employ Opal, a ray-tracing software, alongside Veneris, which uses location-based data from OpenStreetMap to create simulation models. The simulator models are then extended to incorporate transmitter locations given by cellular network tower locations (available from ISED) and receiver locations given by areas of high strategic importance, such as airports, schools, long-term care homes, and hospitals. The simulator is then used to generate power density map defined by the city, cellular network locations, and test measurement points of high strategic importance. To further enhance the granularity of the spatial power density dataset, we investigate the use generative adversarial networks (GANs) conditioned on the transmitter and receiver locations as well as the topographical factors of the city. GANs are particularly well-suited for this task due to their unique image processing and generation abilities and ability to generate realistic data samples and model complex distributions, making them ideal for scenarios with scarce measurements. The anticipated outcomes of this research include the development of synthetic EMF exposure maps that can estimate and validate exposure patterns in urban areas observed using realistic field measurement. These maps will provide valuable insights for the safe and efficient deployment of 5G networks. Ultimately, this study aims to significantly contribute to the body of knowledge on EMF exposure and offer practical solutions for mitigating potential health risks associated with 5G technology.Vaughn Chan
Program: LURA
Supervisor: Suprakash Datta
Profile: Vaughn transferred from the Pure Mathematics program (Bsc) to Engineering (Beng) in order to pursue a career in Software Engineering. He believes AI will profoundly impact the world and thus is interested in getting involved with AI. First, Vaughn will learn Reinforcement Learning (RL) by going through textbooks and tutorials to gain a sense of what RL is. Then, he will apply the concepts to improve wireless communications.
Abstract Title: Reinforcement Learning for Simulated Wireless Mobile Network Management
Abstract Authors: Vaughn Chan, Suprakash Datta
Abstract: Machine learning is a popular artificial intelligence tool for solving problems heuristically instead of brute-force algorithmic solutions. For instance, machine learning can be used for interpreting human communication and tone (via natural language processing) as well as image recognition (via computer vision), both of which would require an insurmountable amount of computer resources to process, which worsens considerably as the scope of the problem increases. One machine learning paradigm is Reinforcement Learning (RL), which works by having an agent interact with its environment to form a strategy to gain as much numerical reward as possible, usually expressed as a Markov Decision Process (MDP). Thus, any problem which can be expressed as an MDP can be optimized via RL. In one such case, it can be used to optimize connections between base stations (BS) and user equipment (UE, such as mobile devices), accounting for limited connection resources across individual BS. The objective of this research is to use RL to equally balance connections to UE across BS within proximity of a BS to maintain a high Quality of Service (QoS). Python is used to load mobile-env as the environment in Gymnasium for setting up a simulation of a wireless mobile network problem. The Stable Baselines module is used to train an RL agent to solve the balancing problem. The viability of RL in solving wireless communication networks is tested by comparing computational resources required to train the agent to the QoS generated, as well as discussion on the feasibility of deploying the agent to an actual mobile network environment. The results of this research are made to encourage the usage of RL in MDP problems in other fields by showcasing its ability to solve an instance of MDP problems.
Xu Nan Shen
Program: USRA
Supervisor: Zhen Ming (Jack) Jiang
Profile: Xu Nan (James) is a 3rd year Computer Science student. He chose to participate in research to learn more about the direction of AI studies and strengthen his resume for future job applications and post-secondary studies. James will be researching the operationalization of RAG-based LLM applications.
Abstract Title: Reducing the Cost of Retrieval-Augmented Generation for Large Language Models by Assessing the Impact of Model Sizes
Abstract Authors: Xu Nan Shen, Peixuan Xia, Zhen Ming Jiang
Abstract: In addressing the critical challenges of hallucination, non-transparent reasoning, and outdated knowledge in the deployment of Large Language Models (LLMs), the Retrieval-Augmented Generation (RAG) framework has gained significant attention. RAG enhances LLM applications by retrieving and incorporating relevant data from external sources that is beyond LLM’s own training data; this enables LLMs to adopt an ‘open book’ strategy and leverage external knowledge to enhance output quality. However, the adoption of RAG escalates both computational and temporal costs associated with LLM usage. One potential solution is to employ smaller models (e.g., GPT3.5) instead of larger ones (e.g., GPT4), when smaller models can generate comparable answers, thereby reducing the cost of RAG applications without degrading performance. In our research, we focus on the question-and-answer scenario and propose a hypothesis: questions can be classified such that larger models are only employed when smaller models are inadequate. We collect responses from RAG applications using different model sizes on an existing question-and-answer benchmark (MultispanQA) and analyze performance disparities. Additionally, we alter the structure and content of a subset of questions to further investigate the semantic and logical complexities that pose challenges to smaller, larger LLMs or both. We manually label a set of questions according to our findings and then use it to train a decision tree model that predicts the optimal LLM for any given question. We demonstrate that this strategy can reduce costs of RAG applications in question-and-answer scenarios. Lastly, we discuss other potential use-cases and propose future research directions to investigate the implementation of a similar cost-reduction approach in such use-cases.
-
Mechanical Engineering
Amanullah Asim, Joaquin Ramirez-Medina
Program: LURA
Supervisor: Alidad Amirfazli
Program: USRA
Supervisor: Alidad AmirfazliProfile: Amanullah, a dedicated Computer Engineering student, has a keen passion for AI and machine learning. Driven by a desire to innovate, he joined the ARIA research project to apply his technical expertise and creativity. With skills in cloud engineering, MERN stack development, and deployment architecture utilizing Docker and Kubernetes, Amanullah is committed to making a unique impact. Through ARIA, he aims to revolutionize research efficiency and accuracy, using AI to develop groundbreaking solutions that align with his professional abilities. Joaquin is a graduating Mechanical Engineering student. With a keen interest in thermofluids sciences and artificial intelligence, Joaquin is passionate about leveraging these fields to drive innovation and solve complex engineering challenges. His participation in research is driven by a desire to deepen his understanding and contribute to advancements in these areas.
Abstract Title: ProfsNotes AI: A Multilayered Approach to Enhancing Student Learning Through an Intelligent Assistant Application
Abstract Authors: Joaquin Ramirez, Amanullah Asim, Alidad Amirfazli
Abstract: In the evolving landscape of educational technology, there is a growing need for comprehensive, accessible tools that can support the student learning process and tackle large class sizes that hinder timely responses and personal attention to student questions. ProfsNotes AI, aim to address this challenge by developing an intelligent student assistant application. The app primary focuses on utilizing the professor’s course content, including lecture content and course syllabi, augmented by Wikipedia and YouTube, to provide a personalised and responsive learning experience. The application employs a three-layered architecture: 1) frontend, 2) API gateway, and 3) microservice layers. The frontend layer handles retrieving and routing user input queries to the API gateway layer and presenting returned information to the user. The API gateway layer manages traffic and communication between frontend and microservices routing user input queries and responses, to and from the proceeding microservices. The microservice layer consists of three main services, including the professors notes service, the Wikipedia service, and the YouTube service. The professor’s notes service utilizes a custom database employing vector and graph datastores to query and retrieve relevant information from professor's course content. The Wikipedia service leverages the Wikipedia database to provide targeted responses from the most relevant Wikipedia article, as well as providing relevant graphics to support the responses. The third service incorporates YouTube videos, analyzing video transcripts to embed relevant clips with precise timestamps, allowing students to directly access exact segments that address their specific questions. We anticipate the integration of the course-specific information with broader knowledge sources is expected to provide a foundational level of understanding of course content for user, as well as foster a deeper level of learning. Future work will focus on refining the system based on user feedback and expanding capabilities over a wider range of academic disciplines.
Basit Ilyas
Program: Mary & Hubert Lynch Undergraduate Research Internship
Supervisor: Pouya Rezai
Profile: Basit Ilyas is an incoming fourth-year student studying Mechanical Engineering at York University. Basit is in the research which is based on lab-on-a-chip electrochemical detection by fabrication and integration of microfluidic channels and electrodes. Basit chose to participate in this research due to a strong background in electrochemistry and microfluidics. Building upon her academic background in fluid mechanics, he aspires to explore the exciting realm of microfluidic devices and contribute to the development of innovative solutions that can revolutionize electrochemical detection, biotechnology, and other related industries.
Abstract Title: Development and Testing of a Microfluidic Electrochemical Based Water Monitoring Device
Abstract Authors: Basit Ilyas, Shapour Jafargholinejad, Pouya Rezai
Abstract: Ensuring the quality of drinking, waste, fresh, and seawater is necessary for human, animal, and plant health. Conventional techniques of water quality monitoring often depend on laboratory-based equipment or expensive, complex portable sensors for on-site analysis, which are time-intensive and require trained personnel. Microfluidics has emerged as an effective alternative, with the potential to transform conventional analytical systems. However, there is a need for the development of a simple, low-cost microfluidic device which is capable of fluid handling, quantification, high sensitivity and mass production. This project aims to accurately detect and quantify water contaminants using a microfluidic electrochemical sensor. Our hypothesis is that this device will provide a reliable and a cost-effective approach to water analysis. The device fabrication involves integrating microfluidic channels with electrodes, which have been tested with various designs to ensure optimal dimensions, efficient sample flow, controlled fluid behaviour, and precise contaminant analysis. The electrode configuration has been optimised to seamlessly integrate with the microfluidic channels, facilitating accurate electrochemical detection and analysis. We have conducted lead detection, including the presence of interference ions, to ensure the device's durability and performance in real-world conditions. Additionally, the device has been tested for biological contaminants, specifically bacteria detection. Our findings demonstrate that the optimised electrode design, when combined with microfluidic channels, enables efficient transfer of electrochemical signals and precise detection and quantification of contaminants, such as heavy metals and bacteria, in water samples. This capability for real-time data collection supports timely decision-making in water resource management, ensuring the provision of safe and clean water to communities. This sensor contributes to United Nations Sustainable Development Goal 6 by ensuring water and sanitation access for all, showcasing microfluidic advancements in environmental monitoring. It protects public health and addresses the demand for efficient, accessible water quality monitoring solutions worldwide.
Bin Xu
Program: LURA
Supervisor: Alidad Amirfazli
Profile: Bin Xu is a 2nd year Computer Science student who has a keen interest in the impact of insects onto Aluminum and Glass Substrates which is his research focus.
Abstract Title: Shape Analysis of Insect Residues
Abstract Authors: Bin Xu, Alidad Amirfazli, Pierre Lesbats
Abstract: The impact of insects on transportation surfaces such as car windshields and motorcycle visors often results in insect splats that can compromise user safety by obstructing the driver’s view. These splats leave complex shapes of insect residues, which present challenges in their classification and characterization. To address this issue, a specialized image processing tool has been developed to effectively analyze and categorize the shapes of these insect residues. The tool uses experimental images of insect residues on a glass with a white background, typically characterized by high contrast and marred by non-uniform background lighting. Image processing techniques, including the Fast Fourier Transform and automatic thresholding for polygon similarity, are utilized to mitigate background colour variations and enhance the clarity of residue outlines. Despite these technological advancements, the analysis process faces significant challenges due to defects and noise within the residue shapes, which can distort critical features and complicate accurate classification. This underscores the ongoing need for further refinement of the tool’s capabilities to achieve more reliable and precise categorization of residue shapes. Current research is not only focused on improving these techniques but also on expanding the tool’s applications. Future developments will test the tool’s effectiveness on different substrates, such as aluminum foil, to evaluate its adaptability and the robustness of the generated outcomes. This work is crucial as it aims to provide a standardized method for classifying insect residue shapes, which has significant implications for improving transportation safety by aiding in the quick and efficient cleaning of critical visibility areas on vehicles.
Chrislord Antiaye
Program: USRA
Supervisor: Solomon Boakye-Yiadom
Profile: Chris is a second year Mechanical Engineering student with a keen interest in Additive Manufacturing at U of T. Chris will be researching High Entropy Alloys produced through Powder Bed Fusion, a type of Additive Manufacturing. High Entropy Alloys are materials with desirable properties such as high resistance to corrosion, high strength, etc.
Abstract Title: An Analysis Of The Process Parameters Of Laser Selective Melting And Their Effects On The Microstructure And Mechanical Properties Of Cantor High Entropy Alloys
Abstract Authors: Chrislord Antiaye, Joseph Agyapong, Solomon Yiadom-Boakye
Abstract: As technology progresses, so does the need for advanced materials with high strength, high toughness, etc. In 2004, unlike conventional alloys, dr. Cantor and his team of researchers combined four or more metallic metals in equal proportions. They called it the high entropy alloy (hea). This new discovery gained considerable attention with its high strength, toughness and resistance to corrosion. The problem being faced now is the means of manufacturing these heas. In recent times, laser selective melting (lsm) has proven to be the way forward. Lsm involves melting and fusing the powdered form of heas to create a solid part. However, little is known about the process parameters and their effect on heas. The purpose of this research is to understand the effects of the process parameters used in lsm on the microstructure and mechanical properties of cantor heas. The process parameters are laser power, scan speed, hatch speed, layer thickness and volumetric energy density (ved). The experiments conducted showed that a slower scan speed and a higher ved significantly increase the density of hea, a higher ved results in a decrease in both micropores and macropores, variations in ved results in different cellular structures, the fracture toughness seems to be affected by the individual process parameters, etc. In the future more research will be conducted to further explore the process parameters in order to tailor them to specific desired properties and microstructures depending on their intended applications. These studies will help manufacture materials to meet technological advancements and help us reach worlds beyond the stars.
Ibrahim Rfifi
Program: LURA
Supervisor: Roger Kempers
Profile: Ibrahim is a third-year mechanical engineering student at York University. Three words that summarize his personality are curious, passionate and dedicated. He is involved with organizations such as Lassonde Motorsports, the Lassonde Academic Honesty Panel and the Lassonde Student Workload Working Group. Some of Ibrahim’s ongoing personal projects include a ball-balancing control system, an electromechanical actuator and a diagnostic toothbrush for Cushing's syndrome, Parkinson's disease and breast cancer. His research interests span thermo-fluid sciences, molecular biology as well as mechatronics and control.
Abstract Title: Forced Convection in Additively Manufactured Mini Channels for Electronics Cooling Applications
Abstract Authors: Ibrahim Rfifi, Mohamed Hasan, Roger Kempers
Abstract: With modern power electronics, effective heat dissipation is essential and mini-channel heat sinks are often used as part of liquid cooling systems to effectively cool high-power components. Mini-channel heat sinks fabricated using additive manufacturing (AM) techniques, such as laser powder bed fusion (LPBF), can offer advantages over traditionally machined counterparts. This includes enhanced geometric complexity and precise customization, as well as cost-efficiency and reduced production times. However, there exists a lack of understanding regarding the thermal-hydraulic performance of liquid on the relatively rough LPBF surfaces. The objective of the present study is to characterize convection heat transfer and pressure drop in LPBF mini channels. The parameters under investigation include five design variations of mini-channel samples fabricated using LPBF, alongside a machined sample serving as a control. The effect of groove height (ranging from 0.5 mm to 1.5 mm) and channel spacing (approximately between 0.4 mm and 0.9 mm) was evaluated. A forced flow apparatus was developed to quantify both the fluid pressure drop and the convection heat transfer coefficient through the different samples over a wide range of flow rates (Reynolds numbers) and heat inputs. The thermal and hydraulic performance of the heat sinks were evaluated across a flow rate range of 0.02 L/min to 0.4 L/min with water as the working fluid. Initial results indicate that the rough LPBF channels can enhance convective heat transfer, however, this comes at the expense of a higher pressure drop compared to their machined channel counterparts. The results from this research will help in the design of more complex LPBF fabricated liquid heat sinks and thermal management technologies to address the increasing demand for effective heat dissipation solutions in modern technologies.
Josue Montero
Program: USRA
Supervisor: Reza Rizvi
Abstract Title: Data-Driven Characterization and Analysis of Automotive Composites using Machine Learning
Abstract Authors: Josue Montero, Hamed Esmaeili, Reza Rizvi
Abstract: This research project aims to advance the characterization and analysis of automotive composites through a data-driven approach using machine learning. The focus is on extracting material properties from non-destructive instrumented indentation experiments and finite element analysis (fea) simulations. Machine learning neural networks (nn) play a crucial role in approximating the inverse function that maps experimental observations to material parameters. This task is particularly challenging when relationships between observations and parameters are non-linear, necessitating extensive datasets for accurate model development. Fea serves as a robust method for generating data used in training nn models, validated by ensuring that simulation results align closely with experimental indentation outcomes for identical materials. While nanoindentation techniques have inherent limitations compared to traditional uniaxial tension tests, which provide comprehensive stress-strain behavior and measure bulk mechanical properties, they offer significant advantages at smaller scales. Nanoindentation requires minimal sample preparation, is non-destructive, and allows for rapid, automated testing. The integration of nanoindentation with machine learning represents a powerful approach to achieve precise, non-destructive testing. This approach not only reduces material waste but also cuts down on the costs and complexities associated with conducting traditional destructive tests like uniaxial tension tests. The research investigates novel methodologies that incorporate previously overlooked parameters to enhance the accuracy of fea simulations within the machine learning framework. Key aspects of the study include data acquisition from experimental tests, rigorous data processing techniques, and the development of sophisticated machine-learning models tailored for predicting mechanical properties of materials. By leveraging advanced computational techniques and experimental data, this research aims to contribute significantly to the understanding and application of automotive composites in engineering and manufacturing contexts.Julia Rodriguez
Program: USRA
Supervisor: Roger Kempers
Profile: Julia is a second year Mechanical Engineering student with an interest in thermal systems and a strong enthusiasm toward environmental stewardship. By participating in research, she hopes to grow her knowledge in her field of interest while contributing to the advancement of sustainable technology. Through this opportunity, Julia plans to gain the necessary experience to pursue further work in automotive innovation.
Abstract Title: Experimental Investigation of Thermal Performance of a Two-Phase Loop Thermosyphon using R1234yf
Abstract Authors: Julia Rodriguez, Roberta Perna, Roger Kempers
Abstract: Two-phase loop thermosyphons are effective heat transport devices used for electronics cooling in a variety of industrial applications. A two-phase loop thermosyphon relies on buoyancy driven flow and gravity to circulate the working fluid as it undergoes phase change, leaving the evaporator outlet to flow through a riser as a partial liquid-vapor mixture, condensing back to liquid in the condenser, and subsequently flowing back down towards the evaporator through the downcomer. Previous research suggests that design refinement of a two-phase loop thermosyphon with r1234yf working fluid can achieve comparable performance to that of r134a, a high global warming potential (gwp) refrigerant. Environmental concerns require the development of a thermosyphon suitable for use with low gwp refrigerants, following the growing use of r1234yf in various heat transport applications, particularly in the automotive industry, due to its low gwp value and zero ozone depletion potential. The objective of this investigation is to design, build, and test an r1234yf two-phase loop thermosyphon and quantify its thermal performance through analysis of system thermal resistance and maximum heating power. The proposed system will consist of an electrically heated, machined aluminum evaporator with a transparent acrylic cover for flow visualization. A stainless steel adiabatic riser and downcomer will be equipped with sight-glasses for further visualization of working fluid phase, while condensation will be facilitated in a water cooled plate heat exchanger. Thermocouples are used to measure temperature throughout the apparatus to quantify thermal resistances. Through the variation of evaporator design, the desired vapor quality at the outlet of the evaporator can be obtained. The optimal evaporator geometry will enable adequate heat transfer efficiency and operational stability of the system at high heat flux conditions. The experimental optimization of a two-phase loop thermosyphon charged with r1234yf can further decrease the environmental impact of essential thermal management systems.
Lexiang Wang
Program: LURA
Supervisor: Alidad Amirfazli
Profile: Lexiang is a third year MECH Eng student who decided to conduct their second coop term to exam their ability and capability after 3 years of study, with an interest in the research topic provided by the supervisor.
Abstract Title: Lunar Dust Mitigation Mechanism Using Polydimethylsiloxane
Abstract Authors: Lexiang Wang, Yating Hu, Alidad Amirfazli,
Abstract: Lunar dust poses significant risks during human explorations on the moon, necessitating the development of effective mitigation methodologies. Previous research has focused on static and cloud lunar dust, ranging from 0.1 to 10 μm, characterized by jagged shapes and high electrical adhesivity. The primary risks associated with lunar dust include increased thermal load, abrasion of critical components, and equipment clogging. Multiple solutions have been proposed and applied over the past decades, including mechanical, fluidal, and electrodynamic/electrostatic methods. To mitigate lunar dust from critical equipment such as cameras and visors, we present a new mitigation mechanism using a Polydimethylsiloxane (PDMS) pad. This non-toxic, relatively adhesive, silicon-based cured polymer lifts dust particles through viscous forces and deformation induced by external forces. Since the performance of the PDMS pad under lunar conditions is undetermined, initial tests will be conducted in Earth environments. Our objective is to use a specially designed apparatus to apply external forces on the PDMS pad, aiming to lift at least 75% of the dust sample. In our study, we examined a uniform layer of static dust sample sized between 0.125 and 0.105 mm. The results were influenced by three main factors: the viscosity and dimensions of the PDMS pad, the particle size and weight of the dust sample, and the applied forces. By controlling these variables and applying a range of vertical forces, we generated multiple data sets and related plots. Our experiments provide pioneering insights into possible lunar dust mitigation solutions, laying the groundwork for future lunar missions. Future experiments will focus on varying the mass of the dust samples while maintaining consistent PDMS viscosity, dimensions, and applied forces to explore the real operational conditions of the PDMS pad. Additionally, the effectiveness of the PDMS pad on cloud dust will be researched.
Luna Marouf
Program: LURA
Supervisor: Marina Freire-Gormaly
Profile: Luna decided to apply to Dr. Marina's research due to her excitement in exploring her program and her interest in the topics that are being researched. Since Luna just finished her first year, she really wanted to get a bit of hands-on experience in the Lab, and work with people of more knowledge and experience to learn and experiment more about one of the fields that she is interested in which is air and water purification. She also thinks that she can really help bring new ideas and findings or just affect the research project that she is currently working on in the best way possible.
Abstract Title: Reverse Osmosis Membrane Coatings to Mitigate Fouling and Improve Water Quality
Abstract Authors: Luna Marouf, Thipphathong Piluk, Robert Liang, Marina Freire-Gormaly
Abstract: According to the World Health Organization, 2.2 billion people globally lack access to clean drinking water as of March 2024 [1], a challenge that Sustainable Development Goal number 6 aims to address. Small-scale renewable-powered reverse osmosis (RO) systems are effective for providing clean drinking water to remote, off-grid communities. RO, a membrane-based desalination method, produces clean water at lower energy costs than thermal desalination methods. However, foulants such as salts, suspended solids, organics, and biological material can cause membrane scaling and fouling, reducing effectiveness and increasing energy requirements. This study polymerized fourteen different RO membrane coatings to evaluate their effectiveness in minimizing membrane scaling. The RO membrane coating of (3-Aminopropyl)triethoxysilane (APTES), Azobisisobutyronitrile (AIBN), Polyacrylic acid (PAA), and Polyvinyl alcohol (PVA) resulted in less fouling, making it longer lasting than the bare RO membrane due to reduced adherence of salt crystals. Characterization was conducted using an experimental lab-scale membrane testing setup with triplicate measurements of membrane permeability, and pre-and post-membrane autopsy using an Atomic Force Microscope (AFM), Drop Shape Analyzer (DSA), and Scanning Electron Microscopy (SEM). DSA results showed this coating increased hydrophobicity compared to all other types. Additionally, this novel membrane coating had a smoother surface with a root mean square roughness (Sq) value of 0.41 ± 0.02 µm as found by the AFM compared to the bare RO membrane and all thirteen coatings. Our novel RO membrane coating of APTES, AIBN, PAA, and PVA reduces scaling and maintains high permeability after long-duration experiments. This novel coating has the best membrane performance quantified using water quality, high hydrophobicity, low roughness, and maintaining high membrane permeability during operation. In the future, this coating can be commercialized and used in RO membranes that are commercially available worldwide, improving the long-term reliability of RO systems and supporting SDG 6.
Sasha Brucker
Program: LURA
Supervisor: Roger Kempers
Profile: Sasha is a 2nd year Mechanical Engineering student. She is passionate about sustainable energy and energy storage systems. She is participating in research to broaden her understanding of key concepts within her degree and to explore subjects within the industry.
Abstract Title: Flow Visualization in Additively Manufactured Two-Phase Heat Transfer Devices
Abstract Authors: Sasha Brucker, Roberta Perna, Roger Kempers
Abstract: With advancements in the electronics industry, thermal management has raised a need for effective electronics cooling systems. Hollow-finned two-phase heat transfer devices facilitate efficient heat transfer between the heat source and heat sink through continuous evaporation and condensation of a working fluid. These devices increase the efficiency of electronics cooling systems and have a wide variety of industrial applications. In previous research, the thermal performance of an additively manufactured, hollow heat sink with an integrated two-phase heat transfer region was investigated. Several wick geometries on the base plate of the device were used to draw acetone to a heat source and the heat sink was tested under natural and forced convection. The objective of the present study is to better understand how the internal two-phase heat transport occurs through flow visualization and to find the relative contribution of each component in the thermal resistance network. Two procedures were utilized to investigate flow visualization. First, a transparent heat sink, manufactured from high temperature resistant resin, was used to directly examine the internal two-phase flow. Design modification of the heat sink must be considered to avoid leaks. Second, an infrared camera was used to map the external surface temperature of the edge of the heat-transfer region of the heat sink. The edge was then painted black to study the two-phase flow of the working fluid with respect to temperature. The results of the tests involving the infrared camera will be used to calculate thermal resistances across the device and support corresponding computational fluid dynamics simulations.
Wilyam Semere Hayelom
Program: USRA
Supervisor: Marina.Freire-Gormaly
Profile: Wilyam is a third-year Mechanical Engineering student, passionate about sustainability and environmental conservation. Participating in Professor Marina's research on clean water and air fascinated him, especially considering the urgent need for such resources in his home country Eritrea as well as Uganda where he grew up in.
Abstract Title: Triplicate Lab Scale RO system
Abstract Authors: Wilyam Hayelom, Felix Bourget, Thipphathong Piluk, Marina Freire-Gormaly
Abstract: Many remote communities around the world lack access to clean drinking water. A possible solution to this problem is the application of a solar photovoltaic reverse osmosis (PVRO) system. The drawback of this system is its large power consumption, as high pressure is needed to pump water through the system's membranes. These membranes are found inside each of the three CF016 flow cells that have a maximum operating pressure of 400 psi to allow flow through and out the permeate line. The permeate line is for filtered water and the concentrate line is for unfiltered water. Over the summer, I have worked with a PhD student to bring this project to life. I am doing this research as it aligns with my interests in providing clean drinking water to my home country in Africa. I have worked with a fellow undergraduate student, Felix Bourget, to control a Hydra Cell pump using a variable frequency drive and allow for water to be pumped to all three flow cells and pass through the membrane, thus being filtered. The system consists of a multitude of sensors such as conductivity sensors, Alicat flow meters at each permeate outlet and one pressure transducer, all monitored through LabView due to the analog nature of the sensors. We have found that optimal permeate flow is achieved when using a single flow cell and a valve at the concentrate line restricting concentrate flow, thus generating pressure and promoting filtration. There is a check valve by the pump outlet to avoid any issues from backflow of water due to the valve closure. This research has given me insight on how to work with pumps, tube fittings, electricity, measuring conductivity and how complex the process of desalination can be.
Wolfgang Becker
Program: LURA
Supervisor: Marina Freire-Gormaly
Profile: Wolfgang is a 2nd year Mechanical Engineering student at Lassonde. He chose to participate in this program (LURA) because it is an excellent opportunity to gain experience in research and design work. Wolfgang's proposed research is a continuation of computational fluid dynamics (CFD) research into the airflow in an airliner's cabin. Specifically, he is looking at aerosol dispersal due to the airflow caused by the cabin ventilation system. His work will entail an experimental validation of the CFD study--verifying that the CFD and reality align and evaluating how closely.
Abstract Title: Characterising Respiratory Aerosol Dynamics in a Boeing 737-200 Aircraft Cabin
Abstract Authors: Wolfgang Becker, Abu Raihan Ibna Ali, Marina Freire-Gormaly
Abstract: The SARS-CoV-2 (COVID-19) pandemic has had a massive effect on our increasingly globalized world, and nowhere is this more evident than in air travel. The pandemic revealed a serious lack of data, especially experimental data, on the character of aerosol dispersal inside aircraft cabins. To mitigate this, we propose to gather experimental data on how exactly aerosols disperse through an airliner cabin—specifically, that of a Boeing 737-200, the general ventilation configuration of which is representative of most narrowbody airliner cabin designs. By collecting aerosol concentration data at multiple seat locations, and over time, we produce an easy-to-read map of the aircraft cabin, showing infection risk as a percentage likelihood of infection. By taking these measurements with various locations of cough injection, and simulating standing, walking, and seated situations, we develop an overall idea of what situations carry the highest risk, informing development of future strategies to improve disease resistance in our air travel, both at the design and operational levels. Furthermore, we compare this data, and fluid dynamic data collected in parallel, with the output of prior CFD work on this subject. This enables us to validate prior simulation work, determining how true-to-life the output is, most importantly in terms of how effective CFD is in predicting the locational infection risk. This research focuses on generating data with a practical meaning, with a direct application to industry. The experiment provides experimental data on both infection risk in their aircraft cabins and the usefulness of the tools currently considered the state of the art.
Yosuf Mohamed
Program: USRA
Supervisor: Pouya Rezai
Profile: Born in Egypt, Yosuf is a Muslim 18-year-old premed in his last year of studying biology. He is interested in his research because he believes it will be something very impactful on people's lives. Part of the reason he wants to go to medical school is so that he can have a positive impact on society and his community. He also works as an Environmental service associate at his local hospital. In his free time, Yosuf loves to play sports and spend time with friends and family.
Yuhui (Michelle) Li
Program: LURA
Supervisor: George Zhu
Abstract Title: Swarm Robotics Approach for Capturing Unknown Uncooperative Targets in Space: a Proof of Concept Experiment
Abstract Authors: Yuhui Li, El Ghali Asri, Zheng H. Zhu
Abstract: Close-proximity operations (CPO) are essential tasks in space robotics, with applications such as docking, berthing, debris removal, satellite servicing, and more. Capturing unknown and uncooperative targets in space is fundamental to these operations. A new research approach involves using a swarm of simpler spacecraft as an alternative to a single complex agent. Swarm robotics is a field inspired by the collective behavior of social insects like ants and bees, where multiple simple robots, or agents, work together to accomplish complex tasks that would be challenging for a single, more complex robot. This approach offers advantages such as redundancy, scalability, and robustness, making it particularly suitable for unpredictable and challenging environments like space. To validate the concept and the specific algorithm for swarm control, this experiment utilizes eight DJI Robomaster S1 robots to simulate spacecraft behavior for capturing unknown targets in 2D. The S1 robots are particularly suited for this task due to their omni-directional wheels, which allow motion that closely mimics the movement of spacecraft in the weightless environment of space. Each robot is equipped with an ultrasonic sensor (Marvelmind super beacon) to obtain real-time global positioning, a monocular camera to determine the global position of targets, and an inertial measurement unit (IMU) to update the agent’s orientation. A central PC sends commands to each robot in parallel, allowing them to communicate with each other and share information about the position of the unknown target. Using decentralized swarm control algorithms, the robots follow commands to capture an unknown target in a 2D plane. Results indicate promising capabilities of swarm robotics in navigating unpredictable space environments, underscoring their potential to enhance future space exploration and defense strategies.Yuxin Su
Program: LURA
Supervisor: Solomon Boakye-Yiadom
Profile: Yuxin is a Computer Science student at Lassonde with an interest in computational modeling and data analysis. Yuxin will utilize advanced CALPHAD-based software for computational modeling and phase diagram analysis to test the compositions and thermodynamic properties of High Entropy Alloys designed by Professor Boakye-Yiadom’s team.
Abstract Title: Enhancing High Entropy Alloy Design through CALPHAD Modeling and Specialized Software Integration
Abstract Authors: Yuxin Su, Solomon Boakye-Yiadom
Abstract: The development and optimization of high entropy alloys (HEAs) represent significant progress in materials science due to their unique properties and diverse applications. Traditional methods of designing HEAs are often time-consuming and inefficient, which makes it necessary to develop advanced computational tools to improve the HEAs design process. This project applies advanced CALPHAD (CALculation of PHAse Diagrams) predictive modeling integrated with specialized software to simplify the design and evaluation process of HEAs. The core of our software is a python-based application. It processes input of conventional alloys, performs thermodynamic calculations, and predicts properties of new materials. The software facilitates the analysis of multi-element alloy compositions and identifies optimal element combinations. By utilizing CALPHAD modeling, this software performs essential calculations to predict the stability, suitability, as well as crystal structures of new materials. Essential calculations include enthalpy of mixing, entropy of mixing, radius asymmetry, enthalpy-entropy ratio, valence electron concentration, and electronegativity difference. Each result will be compared with predefined standards to determine a pass or fail outcome, thereby indicating the likelihood of success in new material design. This approach significantly accelerates research by reducing experimental and computational workload, therefore enables rapid and reliable predictions. It not only enhances the precision and productivity of HEAs design but provides a powerful tool for researchers and engineers to invent new materials with predicted properties. The development of our HEAs software also demonstrates the efficiency and accuracy of the CALPHAD methodology in materials science. Future work will be focused on improving the software's predictive power by integrating additional databases and computational algorithms.